Project Year 2: Research Trip to CUNY and Yale University
Date: | 12 December 2023 |
Author: | Eman Ansari, Katarzyna Kapuścińska, Mekhola Doha & Zofia Dukała |

We, a team of students from University College Groningen supervised by Dr. Muhamed Amin, have recently conducted a study using Machine Learning techniques to model the behavior of transition metal ions involved in catalyzing reactions in metalloproteins. In this study titled ‘Bridging the Coordination Chemistry of Small Compounds and Metalloproteins using Machine Learning’, we examine the intricate relationships between small molecules and extrapolate them to predict the oxidation states and bond lengths of the same metal atoms in larger metalloprotein structures.
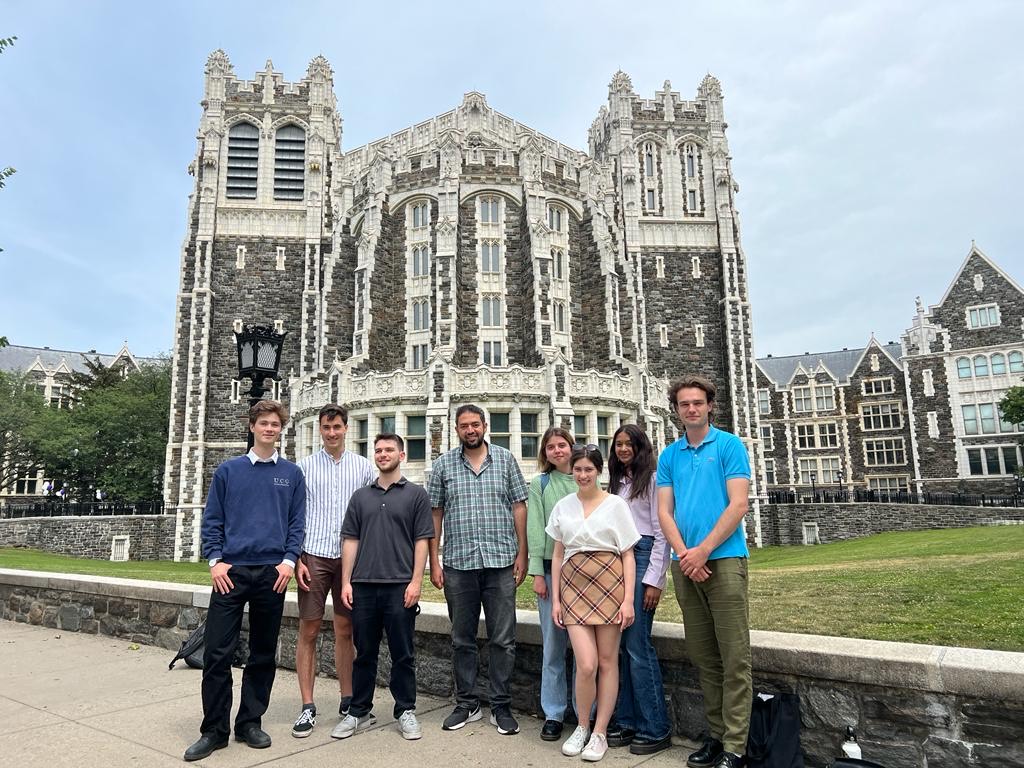
Introduction
Coordination compounds are integral to a wide spectrum of scientific disciplines, such as chemistry, biology, and materials science. These compounds are pivotal in areas like catalysis, medicinal chemistry, and the synthesis of materials. Furthermore, they are essential in biological systems, particularly in metalloenzymes, where they assist in catalytic processes. An in-depth understanding of the principles that govern the formation, structure, and reactivity of these coordination compounds is crucial. Such knowledge is vital not only for enhancing our comprehension of chemical bonding but also for the effective application of these compounds' diverse functionalities in both experimental and practical scenarios.
Research Overview
Metalloproteins require metal ions as cofactors to catalyze specific reactions with remarkable efficiency and specificity. In various electron transfer reactions, metals in the active sites change their oxidation states to facilitate the biochemical reactions. Cryogenic electron microscopy (cryoEM), X-ray and X-ray free electron laser (XFEL) crystallography are used to image metalloproteins to understand the reaction mechanisms. However, radiation damage in cryoEM and X-ray crystallography, and the challenge of generating homogenous crystals and keeping the appropriate experimental conditions for all the crystals in XFEL crystallography, may alter the oxidation states.
Here, we build machine learning models trained on a large dataset from the Cambridge Crystallography Data Center to evaluate the metal oxidation states. The models yield high accuracy scores (from 82% to 91%) for all metals in the small molecules. Then, they were used to predict the oxidation states of more than 30,000 metal clusters in metalloproteins with Fe, Mn, Co and Cu. We found that most of the metals exist in the lower oxidation states and these populations correlate with the intrinsic reduction potentials of the metal ions. Furthermore, we found no clear correlation between these populations and the resolution of the structures, which suggests no significant dependence of these predictions on the resolution. Our models represent a valuable tool for evaluating the oxidation states of the metals in metalloproteins imaged with different techniques. The data files and the machine learning code are available in the supplementary information.
Our experience
Initially, the research required a prerequisite understanding of essential chemistry concepts and as students without a background in chemistry, we found it challenging to navigate the research question and methodology without it. We spent a significant portion of time getting accustomed to the new chemical concepts, in addition to the unfamiliar softwares we had to utilize, such as Pymol, Gaussview and the RUG cluster computers. A highlight of our research project was a trip to the United States, where we engaged with research groups at The City College of New York and Yale University. We had the opportunity to present our preliminary work and exchange ideas with distinguished researchers working in this field who enriched our understanding of the subject by offering their invaluable insights and constructive criticisms. We were exposed to the rigor of the scientific method which thoroughly improved our own conduct as student researchers. Overall this research trip proved to be a pivotal point in our team’s learning curve as a whole and had an extensive impact on the study.
Our student team included Katarzyna Kapuścińska, Zofia Dukała, Mekhola Doha, Eman Ansari, Tristan Timpers, Adam Bahelka, Asher Reeves and Michal Evenhus. We're grateful for the support from the Enhanced Undergraduate Funding of the University of Groningen and the National Institutes of Health (NIH).
For those keen on diving deeper into our findings, the full paper can be found in the Journal of Chemical Information and Modeling and our machine learning code is available in our public GitHub repository.
About the author
Eman Ansari, Katarzyna Kapuścińska, Mekhola Doha and Zofia Dukała are undergraduate students in Liberal Arts and Sciences at UCG. In 2023, they collaborated in a project together with research groups from Yale University and NIH, leading to a publication in the Journal of Chemical Information and Modeling.