In-house expertise
Our multidisciplinary research centre integrates expertise in materials science, physics, device engineering, mathematics, computer science, artificial Intelligence, electrical engineering and robotics. This expertise is embedded in three different institutes within the Faculty of Science and Engineering at the University of Groningen: the Zernike Institute for Advanced Materials, the Bernoulli Institute for Mathematics, Computer Science and AI and the Engineering and Technology Institute Groningen (ENTEG).
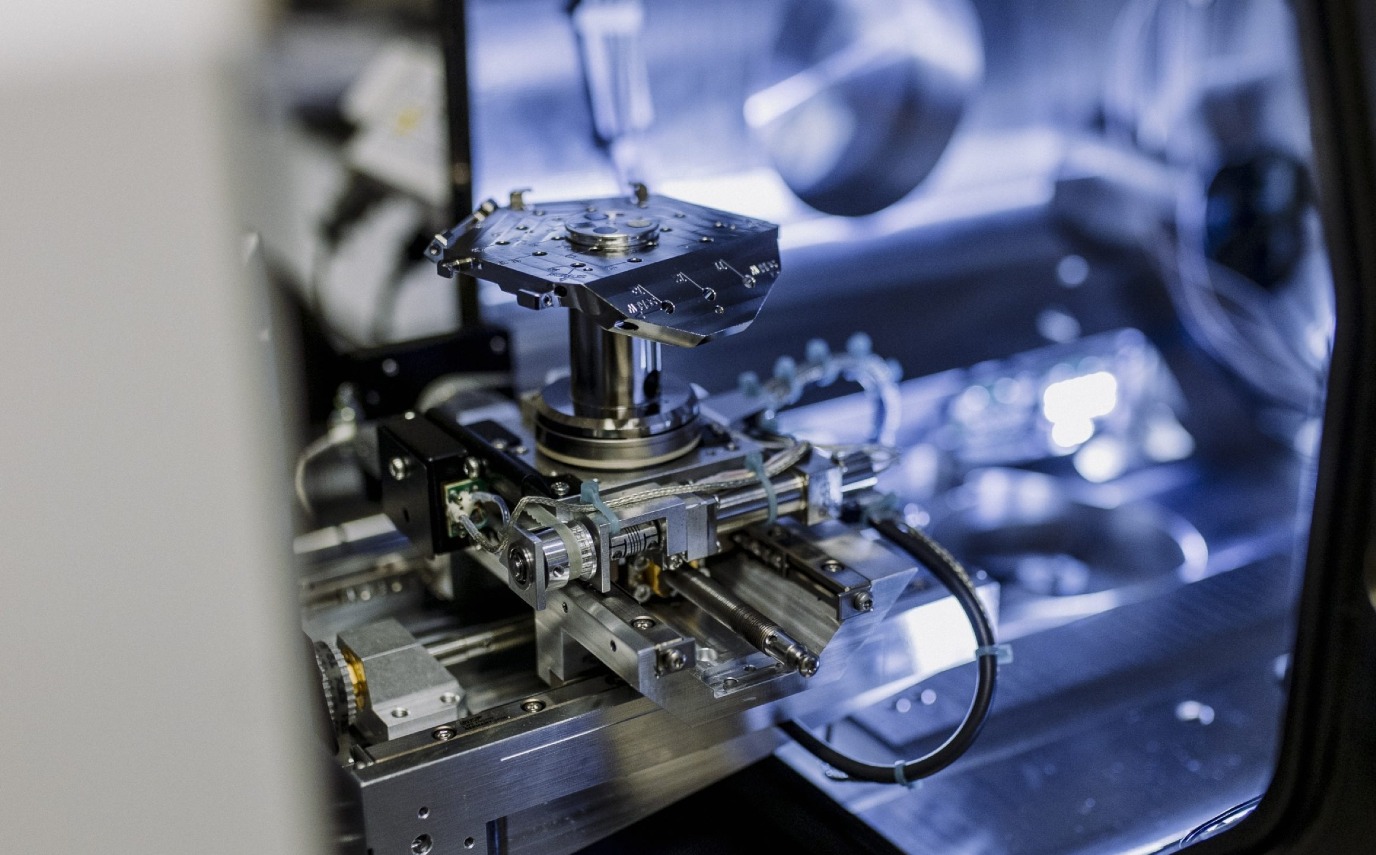
Advancing neuromorphic computing
Leveraging strengths in neuromorphic computing and brain-inspired computing, our unique approach focuses on developing scalable neuromorphic chips and neural network hardware. This scalability allows us to surpass current solutions, driving advancements in neuromorphic processors and AI.
Since this is a very new field, its future will strongly depend on the quality of our education. Find out more about the student courses that CogniGron offers.
Research disciplines and experts
Materials Science
At the heart of our research is the advancement of materials that enable rapid signal transfer and long-term learning effects, akin to memristors. We focus on developing materials with adaptive features and low-energy consumption, aiming to create systems that integrate both short-term signal transfer and long-term learning dynamics. This involves exploring various material systems and devices, from nanoscale materials with intrinsic properties to hybrid and fully integrated low-power devices, drawing on our expertise in tuneable conducting domain walls, skyrmions, and optoelectronics.
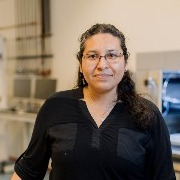
Prof. Mónica Acuautla
Materials for Mechatronics
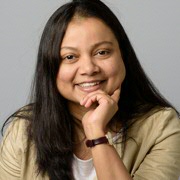
Prof. dr. Tamalika Banerjee
Spintronics of Functional Materials
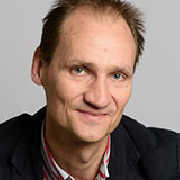
Prof. dr. ir. Bart Kooi
Nanostructured Materials and Interfaces
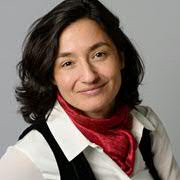
Prof. dr. Maria Loi
Photophysics and OptoElectronics
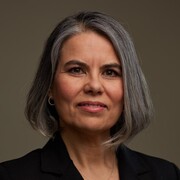
Prof. dr. Beatriz Noheda
Nanostructures of Functional Oxides
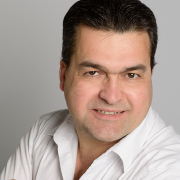
Prof. dr. George Palasantzas
Physics (Surface interactions and Nanostructures)
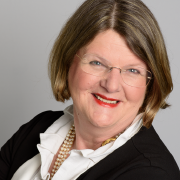
Prof. dr. Petra Rudolf
Experimental Solid State Physics
Artificial Intelligence
The University of Groningen excels in artificial intelligence, pattern recognition, machine learning, and cognitive modelling, with expertise spanning neural networks in both artificial and natural systems, cognitive neuroscience, and data science engineering. Recent advancements in Deep Learning have revolutionised machine learning, enabling neural networks to handle complex 2D and 3D patterns with greater efficiency and robustness. This progress in Cognitive Computing facilitates the detection and prediction of spatiotemporal patterns from raw data. However, challenges remain, such as the high energy consumption of current neural-network implementations compared to the brain’s efficiency. To address these issues, we aim to explore new materials and computing paradigms suitable for neuromorphic computing, leveraging both theoretical and practical approaches. Collaboration with experts at CWI in Amsterdam and the University of Waterloo will support our efforts to develop models and methods for efficient, low-energy neural computing.
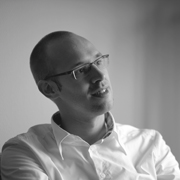
Prof. dr. Jelmer Borst
Computational (Cognitive) Models
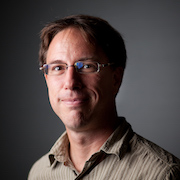
Prof. dr. Matthew Cook
Cortex-inspired Computing
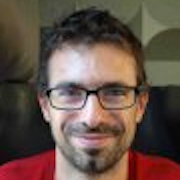
Prof. dr. Davide Grossi
Cognitive Multiagent Systems
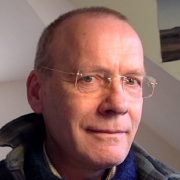
Prof. dr. Herbert Jaeger
Computation in Cognitive Materials
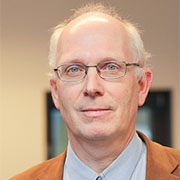
Prof. dr. Lambert Schomaker
Artificial Intelligence
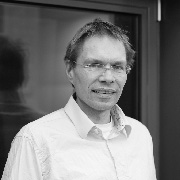
Prof. dr. Niels Taatgen
Artificial Intelligence
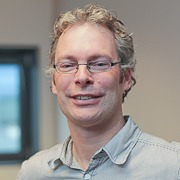
Prof. dr. Bart Verheij
Computational argumentation
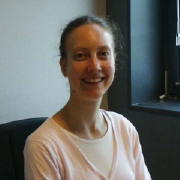
Dr. Marieke van Vugt
Cognitive Modeling
Mathematics
The University of Groningen’s mathematics expertise covers a broad range, including Statistics & Stochastics, Systems & Control, Computational Mathematics, and Dynamical Systems. This expertise is closely connected to computer sciences, particularly through Systems & Control, which is vital for modelling large-scale stochastic systems and performing complex simulations. The department’s focus includes the analysis and control of dynamic systems using mathematical theories from analysis, algebra, geometry, and measure theory.
Key areas of interest involve studying both long-term and transient behaviours, bifurcations, and employing numerical and visualisation tools. Additionally, the department highlights algorithmic and constructive methods, especially in statistical mechanics and high-dimensional inference. There are ample opportunities for collaboration between mathematics and materials engineering, integrating fundamental research with practical applications in engineering and the natural sciences.
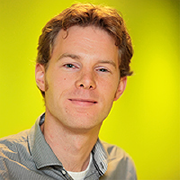
Prof. dr. Bart Besselink
Systems and Control Theory
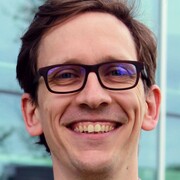
dr. Gilles Bonnet
Stochastics
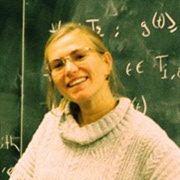
Dr. Serte Donderwinkel
Probability Theory
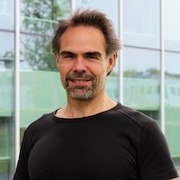
Prof. dr. Marco Grzegorczyk
Computational Statistics
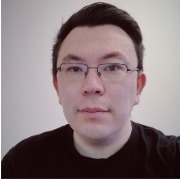
Dr. Hildeberto Jardon Kojakhmetov
Mathematics, Interdisciplinary Applications
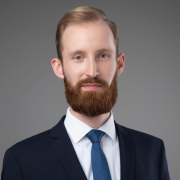
Dr. Julian Koellermeier
Computational Mathematics
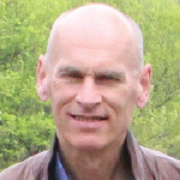
Prof. dr. Arjan van der Schaft
Applied Analysis
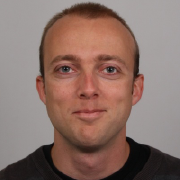
Dr. Alef Sterk
Dynamical Systems Theory
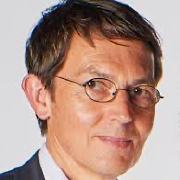
Prof. dr. Holger Waalkens
Dynamical Systems Theory
Computer Science
The computer science department at the University of Groningen is essential to CogniGron, with its strong expertise in Image Processing & Computer Vision, which is perfect for creating advanced algorithms to analyse images of nanomaterials. The department’s strengths in biologically inspired pattern recognition and machine learning align closely with Cognitive Computing. Additionally, expertise in Computer Graphics, Visualisation, and Visual Analytics aids in designing and understanding cognitive materials and complex systems. Knowledge in Fundamental Computing, including logic, algorithms, and data structures, is crucial for developing new computing paradigms for cognitive systems. Collaborative efforts with materials scientists focus on using these technologies for efficient image analysis and system design, integrating machine learning and pattern recognition to enhance cognitive system development.
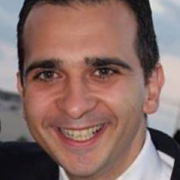
Prof. dr. George Azzopardi
Computer Science
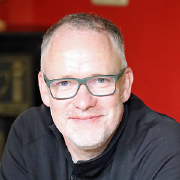
Prof. dr. Michael Biehl
Intelligent Systems
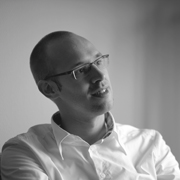
Prof. dr. Jelmer Borst
Computational (Cognitive) Models
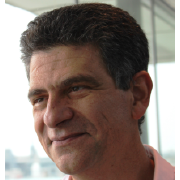
Prof. dr. ir. Georgi Gaydadjiev
Innovative Computer Architecture
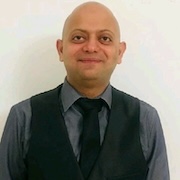
Dr. Farhad Merchant
Innovative Computer Architecture
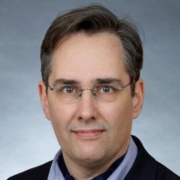
Prof. Dirk Pleiter
Innovate Computer Architectures
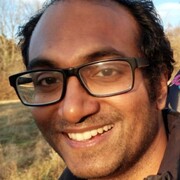
dr. Revantha Ramanayake
Theory of Computation
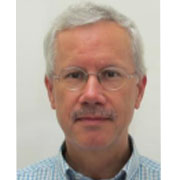
Prof. dr. Jos Roerdink
Scientific Visualization and Computer Graphics
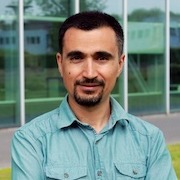
Prof. dr. Fatih Turkmen
Computer and Network Security
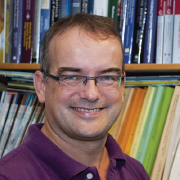
Dr. Michael Wilkinson
Digital image analysis and computer vision
Electrical Engineering and Robotics
At CogniGron, the Electrical Engineering and Robotics discipline is dedicated to advancing neuromorphic computing by developing bio-inspired circuits and systems that emulate the brain's efficiency and adaptability. Our research focuses on creating autonomous robots and complex systems capable of self-learning and decision-making. We aim to revolutionize computing technologies with energy-efficient, compact and robust solutions especially suitable for edge-computing and robotic applications.
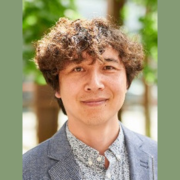
Prof. Ming Cao
Multi-agent systems, autonomous robots, complex systems and networks
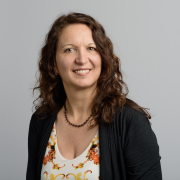
Prof. dr. Elisabetta Chicca
Bio-Inspired Circuits and Systems
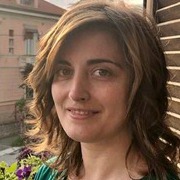
Dr. Erika Covi
Cognitive Devices
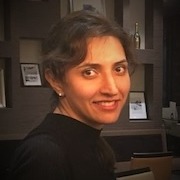
Dr. Bahar Haghighat
Multi-Robot Systems, Mechatronic Design, Multi-Level Modeling, Control
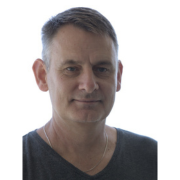
Prof. dr. ir. André van Schaik
Neuromorphic Engineering and Computational Neuroscience
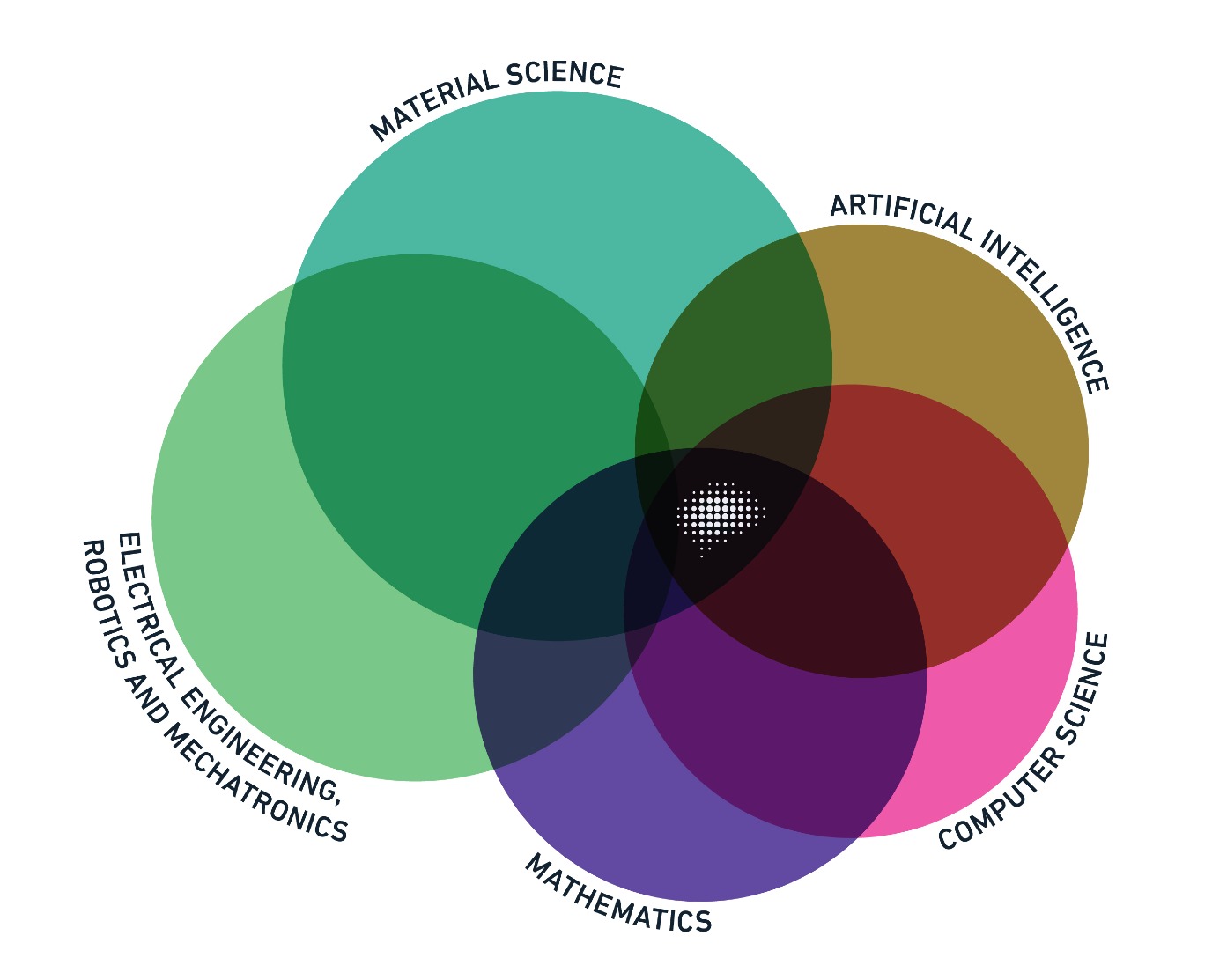
Research projects
Below is an overview of our ongoing research projects, including EU-funded collaborations across materials science, device engineering, and computing systems.
EU projects
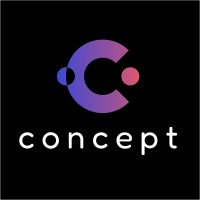
Crystalline Oxides for Next Generation Computing and Emerging Photonic Technologies (CONCEPT)
-
Acronym: CONCEPT
-
Grant Agreement: 101135946
-
CogniGron Participant: Beatriz Noheda
-
Programme: HORIZON-CL4-2023-DIGITAL-EMERGING
-
Duration: 1 Jan 2024 – 31 Dec 2027
-
Links: Project Website · CORDIS
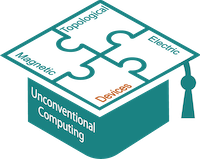
Topological Solitons in Ferroics for Unconventional Computing (TOPOCOM)
-
Acronym: TOPOCOM
-
Grant Agreement: 101119608
-
CogniGron Participant: Beatriz Noheda
-
Programme: HORIZON-MSCA-2022-DN
-
Duration: 1 Sep 2023 – 31 Aug 2027
-
Links: Project Website · CORDIS
Transforming IoT Sensory Systems for a Sustainable Future (SWIMS)
-
Acronym: SWIMS
-
Grant Agreement: 101119062
-
CogniGron Participant: Elisabetta Chicca
-
Programme: ERC Synergy
-
Duration: 1 Jan 2024 – 31 Dec 2029
-
CORDIS: Link
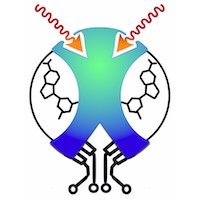
Insect-Brain Inspired Neuromorphic Nanophotonics (InsectNeuroNano)
-
Acronym: InsectNeuroNano
-
Grant Agreement: 101046790
-
CogniGron Participant: Elisabetta Chicca
-
Programme: HORIZON-EIC-2021-PATHFINDEROPEN
-
Duration: 1 Apr 2022 – 31 Mar 2026
-
Links: Project Website · CORDIS
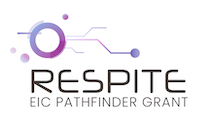
Reconfigurable Superconducting And Photonic Technologies Of The Future (RESPITE)
-
Acronym: RESPITE
-
Grant Agreement: 101098717
-
CogniGron Participant: Bart Kooi
-
Programme: HORIZON-EIC-2022-PATHFINDEROPEN
-
Duration: 1 Mar 2023 – 28 Feb 2027
-
Links: Project Website · CORDIS
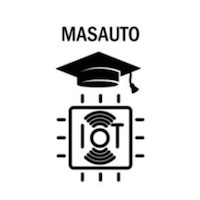
MAterials for Smarter AUTonomous sensOrs (MASAUTO)
-
Acronym: MASAUTO
-
Grant Agreement: 101168161
-
CogniGron Participant: Beatriz Noheda
-
Programme: HORIZON-MSCA-2023-DN
-
Duration: 1 Mar 2025 – 28 Feb 2029
-
Links: Project Website · CORDIS
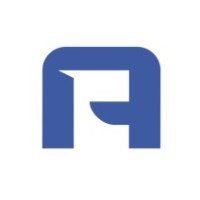
ARCHitectures based on Unconventional Accelerators for Dependable / EnergY-EfficienT AI Systems (ARCHYTAS)
-
Acronym: ARCHYTAS
-
CogniGron Participant: Erika Covi
-
Programme: European Defence Fund (EDF-2023-RA)
-
Awarded: 16 May 2024
-
UG Amount: €817,165.00
-
Links: EDF Project Info · LinkedIn Page
In-Materio Reservoir Computing Using Tailored Epitaxial Complex Oxides (RECOMPUTE)
-
Acronym: RECOMPUTE
-
Grant Agreement: 101203197
-
CogniGron Participant: Martin Sarrott
-
Programme: HORIZON-MSCA-2024-PF
-
Duration: 1 Apr 2025 – 31 Mar 2027
-
Amount: €217,076.16
-
CORDIS: Link
Universality in Phase Transitions in Random Graphs (GraPhTra)
-
Acronym: GraPhTra
-
Grant Agreement: 101211705
-
CogniGron Participant: Serte Donderwinkel
-
Programme: HORIZON-MSCA-2024-PF
-
Duration: 1 Mar 2025 – 28 Feb 2027
-
Amount: €217,076.16
-
CORDIS: Link
MALEFICENT
-
Acronym: MALEFICENT
-
CogniGron Participant: Fernando M. Quintana Velázquez
-
Programme: HORIZON-MSCA-2024-PF
-
Details: Start date and amount to be confirmed
-
CORDIS: (Pending)
Other international projects
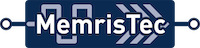
Memristive Time Difference Encoder (MemTDE)
-
Acronym: MemTDE
-
Grant Agreement: 422738993
-
CogniGron Participant: Elisabetta Chicca
-
Programme: DFG
-
Website: MemTDE – MemrisTec
Last modified: | 14 May 2025 3.30 p.m. |