Research Matthias Heinemann
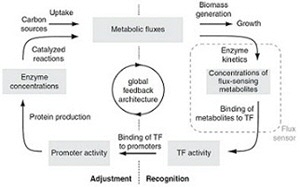
Cellular metabolism with its more than 1000 different chemical reactions is highly complex, and despite decades of research we are still far from understanding the functioning of metabolism. Yet, such understanding is crucial for biotechnology as well as for health and disease, where we start to realize that a faulty metabolism might at the onset of diseases such diabetes, cancer, and of the pathogenicity of microbes, etc. To this end, we aim to generate a systems-level understanding of carbon metabolism. Specifically, we are interested in questions such as (i) what are the intracellular fluxes, (ii) what are the regulation mechanisms that a cell uses to control its metabolic fluxes, (iii) what are the principles underlying metabolism.
At the moment, we are highly interesting in the following specific problems:
- metabolism and bacterial persistence
- metabolism and the eukaryotic cell cycle
- engineering metabolism for bioprocesses
In the last years, for example, we uncovered - first by computational predictions and later validated by experiments - that cells have mechanisms in place that sense metabolic flux and that are used for flux-dependent regulation. Further, we found that flux-sensing and flux-dependent regulation can lead to responsive diversification of clonal E. coli populations into multiple phenotypes, where one of these can be the medically relevant persister phenotype. Very recently, using dynamic single-cell metabolite measurements, we found that metabolism of yeast is an autonomous oscillator, driving the cell through the cell cycle in a flux-dependent manner.
In all projects, we combine experimental and computational (modeling) efforts in an iterative interplay. Where necessary, we develop our new experimental or computational tools. To facilitate the systems biology approach, the group members have diverse educational backgrounds ranging from biology via engineering to computer science. We find such a multidisciplinary environment most stimulating and also most adequate to jointly solve the exciting problems we are looking at.
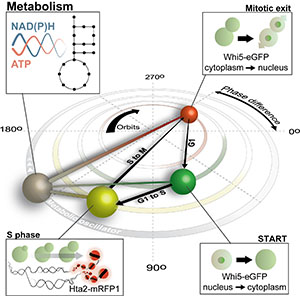
Our research is primarily curiosity-driven, i.e. the main source of motivation comes from the mere interest of getting a deeper insight into metabolism. However, in case one of our generated fundamental findings can, for instance, help improving bioprocesses, we also try to implement this in the applied context.
Currently, we are primarily working with the two model organisms E. coli and yeast. However, as we are not per se primarily interested in these organisms but rather in the underlying metabolic principles, we are also open to look into other organisms. In fact, the long-term vision of the group is to transfer the knhowledge generated with the simple bacterium E. coli and the lower eukaryote S. cerevisiae at some point in the future to the level of mammalian cells.
Selected papers
Papagiannakis A, Niebel B, Wit EC, Heinemann M (2017) Autonomous metabolic oscillations robustly gate the early and the late cell cycle, Molecular Cell, doi:10.1016/j.molcel.2016.11.018.
Radzikowski JL, Vedelaar S, Siegel D, Ortega AD, Schmidt A, Heinemann M (2016) Bacterial persistence is an active σS stress response to metabolic flux limitation. Molecular Systems Biology. 12(9): 882, 10.15252/msb.20166998.
Schmidt A, Kochanowski K, Vedelaar S, Ahrne E, Volkmer B, Callipo L, Knoops K, Bauer M, Aebersold R, Heinemann M (2016) The quantitative and condition-dependent Escherichia coli proteome. Nature Biotechnology, 34: 104-111.
Janssens G, Meinema A, González J, Wolters K, Schmidt A, Guryev V, Bischoff R, Wit E, Veenhoff L, Heinemann M (2015) Protein biogenesis machinery is a driver of replicative aging in yeast. eLife. 10.7554/eLife.08527.
Kotte O, Volkmer B, Radzikowski JR, Heinemann M (2014) Phenotypic bistability in Escherichia coli's central carbon metabolism. Molecular Systems Biology. 10, 736.
Kochanowski K, Volkmer B, Gerosa L, Haverkorn van Rijsewijk BR, Schmidt A, Heinemann M (2013) Functioning of a metabolic flux sensor in Escherichia coli. Proceedings of the National Academy of Sciences of the United States. 110, 1130-1135.
Schütz R, Zamboni N, Zampieri M, Heinemann M, Sauer U (2012) Multidimensional optimality of microbial metabolism. Science, 336, 601-604.
Lee SS, Avalos Vizcarra I, Huberts DHEW, Lee LP, Heinemann M (2012) Whole lifespan microscopic observation of budding yeast aging through a microfluidic dissection platform. Proceedings of the National Academy of Sciences of the United States, 109: 4916–4920.
Last modified: | 26 January 2022 12.15 p.m. |