Research Andreas Milias
The content of this page is outdated. For an up-to-date overview of the our group's activity, please visit https://sites.google.com/rug.nl/milias-argeitis-lab
Our research interests lie on the interface of mathematics, computation and biology. One of our primary theoretical interests has been the learning of dynamical models of biological networks from experimental data. This area includes the problems of parameter inference for various types of models and data, model selection/invalidation [1] and network structure inference. We are particularly interested in addressing biological questions that give rise to these types of problems by adapting and using existing machine learning and statistical approaches, or by developing new methods.
We also maintain a strong interest in problems related to the analysis [2] and simulation of the generated models, to maximize their utility and scope. Our current work is focused on the development of efficient and scalable uncertainty quantification methods for deterministic and stochastic models of biochemical networks. Uncertainty in the predictions made by these systems is typically a consequence of uncertain parameter values, interactions or inputs. Understanding how the different model elements contribute to the uncertainty of the measured outputs, can be of great help in the design of informative experiments [3].
To unravel the complexity of biochemical networks experimentally, we would like to harness the power new molecular tools for delivering perturbations to biological systems, such as optogenetics [4]. Optogenetic tools offer unprecedented capabilities to induce fast, targeted and reversible perturbations to cellular systems, and can thus become an indispensable tool for dissecting complex cellular mechanisms [5]. On the experimental side, the field is currently undergoing very fast growth, as the photochemistry of many different classes of photosensitive protein domains has already been characterized and is being put into use for the construction of optogenetic tools [4]. With these tools, a very large range of cellular functions can already be put under light control: among others, gene expression, protein signaling activity, localization, polymerization, organelle positioning, cell motility and ion channel gating.
The ability to target individual nodes in a cellular network with exquisite precision and time resolution can give rise to new, more powerful approaches for network discovery, model discrimination/invalidation and parameter inference. Currently used computational tools in these areas have been largely influenced by the limited capabilities offered by classical chemical and genetic perturbations and are thus not able to fully realize the potential of optogenetic stimulation. The development of new tools should take into account the availability and features of the controllable input used [6], and optimize the stimulation for the task at hand (e.g. maximally discriminate between alternative models of the system). Embedding the controlled system into a feedback control loop [7, 8] can also enable the use of adaptive control techniques. These approaches can potentially extract more information on the system of interest in an efficient way and thus resolve ambiguities that plague the field of model selection in systems biology. A long-term goal of our research would therefore be to creatively combine optogenetic, machine learning and control theory methods to answer biological questions related to the structure and function of gene and signaling networks.
Cell cycle commitment unraveled by single-cell microscopy, modeling and optogenetics
Cells across all kingdoms of life need to maintain tight control on their division process. In particular, the decision of cells to initiate a round of division is a ubiquitous and essential process, whose dysregulation can dramatically affect cell viability in unicellular organisms, while contributing to carcinogenesis in multicellular organisms such as humans. In budding yeast (Saccharomyces cerevisiae), the short interval in late G1 where commitment to cell division is decided, is known as START. Despite years of research, it is still not clear how the transition through START is triggered, and several conflicting hypotheses have been proposed. On the other hand, microfluidics and single-cell microscopy have opened up new avenues for investigating START at the single-cell level. Moreover, the rapid development of optogenetic tools in the last few years offers the possibility to apply dynamic single-cell perturbations, with great potential for untangling complex intracellular networks.
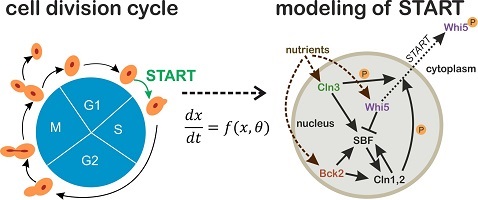
By combining single-cell time-course data on key components of the START network with targeted, reversible and dynamic perturbation of their activities using optogenetic tools, the aim of this project is to unravel the mechanisms that trigger START in budding yeast and to quantitatively describe these processes in a mathematical model. The results of this project will thus provide new fundamental knowledge on cell cycle regulation which, thanks to the high degree of conservation among eukaryotes, will be transferrable to other organisms as well.
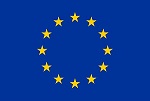
This project has received funding from the European Union’s Horizon 2020 research and innovation programme under the Marie Sklodowska-Curie grant agreement No 798488
References
[1] A. Milias-Argeitis, A.P. Oliveira, L. Gerosa, L. Falter, U. Sauer and J. Lygeros. “Elucidation of genetic interactions in the yeast GATA-factor network using Bayesian model selection”, PLoS Computational Biology, vol. 12, no. 3, 2016
[2] A. Milias-Argeitis, S. Engblom, P. Bauer and M. Khammash. “Stochastic focusing coupled with negative feedback enables robust regulation in biochemical reaction networks”, Journal of the Royal Society Interface, vol. 12, no. 113, 2015
[3] J. Ruess, A. Milias-Argeitis, and J. Lygeros. “Designing experiments to understand the variability in biochemical reaction networks”, Journal of the Royal Society Interface, vol. 10, no. 88, pp. 20130588, 2013
[4] Schmidt, D., and Cho, Y. K. “Natural photoreceptors and their application to synthetic biology”, Trends in Biotechnology, vol. 33, no. 2, pp. 80–91, 2015
[5] Toettcher, J. E., Voigt, C. A., Weiner, O. D., and Lim, W. A. “The promise of optogenetics in cell biology: interrogating molecular circuits in space and time”, Nature Methods, vol. 8, no. 1, pp. 35–38, 2011
[6] J. Ruess, F. Parise, A. Milias-Argeitis, M. Khammash and J. Lygeros, “Iterative experiment design guides the characterization of a light-inducible gene expression circuit”, Proc. Natl. Acad. Sci., USA, vol. 112, no.26, pp. 8148-8153, 2015
[7] A. Milias-Argeitis, S. Summers, J. Stewart-Ornstein, I. Zuleta, D. Pincus, H. ElSamad, M. Khammash, and J. Lygeros. “In silico feedback for in vivo regulation of a gene expression circuit”, Nature Biotechnology vol. 29, no. 12, pp 1114-1116, 2011
[8] A. Milias-Argeitis, M. Rullan Sabater, S.K. Aoki, P. Buchmann and M. Khammash. “Automated Optogenetic Feedback Control for Precise and Robust Regulation of Gene Expression and Cell Growth”, Nature Communications vol. 7, 2016
Last modified: | 09 March 2022 1.35 p.m. |