Schomaker, prof. dr. Lambert
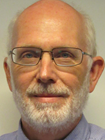
Lambert Schomaker is hoogleraar Kunstmatige Intelligentie en wetenschappelijk directeur van het onderzoeksinstituut ALICE (Artificial Intelligence & Cognitive Engineering). Schomaker heeft zijn naam verbonden aan diverse projecten waarin in schuinschrift geschreven teksten online worden geanalyseerd op basis van de kennis over de bewegingen die bij het schrijven worden gemaakt. Op dit moment werkt hij aan een aantal projecten op het gebied van verwerking op basis van afbeeldingen, online en offline handschriftherkenning, forensische auteursherkenning en cognitieve robotnavigatiemodellen. Zijn werk op het gebied van neurale netwerken voor handschrift- en gebaarherkenning was een voorloper van de hedendaagse methoden van handschrift- en gebaarherkenning die op tabletcomputers zoals de iPad worden gebruikt.
Momenteel werkt hij aan een project (Target) op het gebied van grootschalige opslag, supercomputers en datamining, aan de hand waarvan de generieke zoekmachine Monk voor handgeschreven historische archieven kan worden geïmplementeerd. Het systeem achter Monk is wereldwijd uniek dankzij zijn enorme schaal, genericiteit en het gebruik van ‘live’ , '24/7', machinaal leren. Binnen een ander project, genaamd Mantis, is Schomaker bezig met het ontwikkelen van slimme systemen die problemen in productielijnen kunnen opsporen en oplossen. Schomaker ontwikkelt op basis van de robuustheidsbeginselen uit de Kunstmatige Intelligentie slimme systemen die problemen in productielijnen kunnen opsporen en oplossen.
In 2021 ontdekten prof. Mladen Popović (expert op het gebied van de Dode Zeerollen), promovendus Maruf Dhali en Schomaker, door analyse van het handschrift met kunstmatige intelligentie, dat de beroemde grote Jesaja-rol door twee schrijvers is geschreven.
In 2023 kreeg het project HAICu, waar Schomaker penvoerder vanuit de RUG van is, een subsidie van 10,3 miljoen euro van de Nationale Wetenschapsagenda. In het onderzoeksproject werken AI- en Digital Humanities-onderzoekers samen met erfgoedprofessionals en geïnteresseerde burgers aan wetenschappelijke doorbraken om grootschalige digitale erfgoedcollecties te ontsluiten, te koppelen en te analyseren.
Eerder in het nieuws
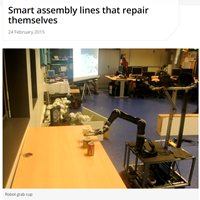
Contact en meer informatie
-
Zie de profielpagina
Publicaties
2025
2024
2023
2022
2021
2020
2019
2018
2017
Laatst gewijzigd: | 02 mei 2025 11:20 |