Instagram teaches AI to recognize rooms
It is not hard for humans to recognize an indoor environment, but teaching an artificial intelligence (AI) system to distinguish an office from a library is. AI systems are usually trained to use images only, and recognizing a space just by looking at objects can easily go wrong. That is why computer scientist Estefanía Talavera Martínez added a new data modality, audio/sound, to the teaching material that the AI system looks at. This resulted in a high success rate in recognizing indoor spaces, and in a new dataset of real-world videos to use in research. Her work was published in the journal Neural Computing and Applications on 22 January.
Estefanía Talavera Martínez is interested in developing algorithms for the automatic analysis of human behaviour. In previous work, she relied on photo streams gathered by wearable cameras to gain an understanding of people’s daily behaviour. These images were first analysed using AI systems. Doing the same with video is a next step, and one with more applications. ‘This could also be used to help robots find where they are, or to monitor the elderly, for example,’ explains Talavera Martínez. However, this requires an automated system that can identify indoor spaces.
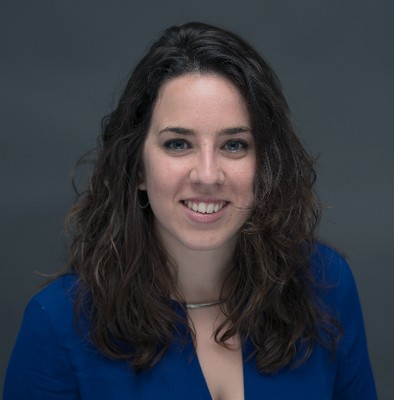
Speech
Previous attempts to teach AI to recognize indoor spaces have not been very successful. ‘One of the reasons for this is that most systems are trained using just one modality, usually recognition of objects in a room.’ Therefore, Talavera Martínez decided to train her system using a second modality: transcribed texts of speech recorded in the videos.
She used real-world videos from Instagram to train her AI system. This was achieved using the images and speech. The spoken texts were transcribed using standard Google speech recognition software. Talavera Martínez and her then Master’s student Andreea Glavan tried different approaches in combining information from images and audio, to find which approach would produce the best result. This resulted in a system that could recognize videos from nine different types of indoor spaces with a 70 percent accuracy, which is higher than previously published systems managed. ‘Tests that we performed confirmed that using this combination results in a better performance of this system than training it using only images or text,’ says Talavera Martínez.
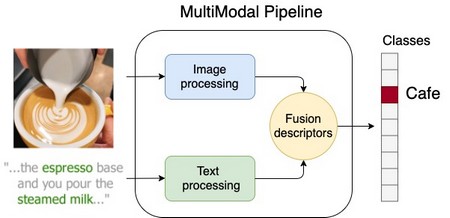
Behaviour
Furthermore, the research project has produced a dataset of 3,788 Instagram videos describing nine indoor scenes. Also, a selection of 900 YouTube videos was used to confirm the results of the training program. ‘We have made both datasets publicly available, the first of their kind.’
Talavera Martínez would like to use the new AI system to further analyse human behaviour from videos: ‘They contain a lot of information, both as individual frames and as sequences. Importantly, our new system would be able to recognize the type of environment in which the images were made.’
Apart from studying behaviour, the system could be used, for example, to monitor patients with a special focus on healthy ageing. It could also be used to identify positive experiences to be relived by people. ‘And we know that people often have a very subjective view of their own life. Our system could provide them with an objective registration and analysis.’
Reference: Andreea Glavan & Estefanía Talavera: InstaIndoor and multi-modal deep learning for indoor scene recognition. Neural Computing and Applications, 22 January 2022.
Last modified: | 07 February 2025 12.07 p.m. |
More news
-
02 July 2025
€ 30 million investment for UG spin-off Portal Biotech
Portal Biotech, a pioneer in nanopore-based protein identification and sequencing technology, has raised € 30 million in Series A funding. The funding will support commercial rollout and team growth.
-
01 July 2025
‘Give seals space’
The Wadden Sea is constantly changing. Native animals need to be able to adapt in order to thrive in an environment that is shaped by the tides. By conducting research on seals in the area, PhD students Margarita Méndez-Aróstegui and Beatriz...
-
30 June 2025
David Lentink partners international research project on animal navigation
Prof. David Lentink is a partner in the NaviSense project awarded 54.7 million euros by the German government to research the mechanisms animals use to navigate and how these mechanisms can inspire technology.