Artificial Intelligence
The University of Groningen holds a strong position in artificial intelligence (knowledge-based and multi-agent systems), pattern recognition/machine learning and cognitive modelling/data-science engineering. We have expertise in neural networks, both in artificial and natural systems, in cognitive neuro-scientific modelling and computational neuroscience. Problems in cognitive systems range from the materials of the computing substrate to high-level cognitive mechanisms and applications.
In recent years, the availability of large data sets and computing power have led to a revolution in machine learning, notably in the area of ‘Deep Learning’ with neural networks (Bengio, 2009; Bengio et al., 2015). Improvements in learning algorithms now allow for neural networks with more layers and parameters (weights) than ever before. For patterns in 2D or 3D arrays, the use of convolution kernels allows a saving in the number of coefficients and a gain in terms of robustness against pattern variation. The concept of a ‘universal function approximator’ has been mathematically supported (Cybenko, 1989; Hornik, 1999).
This state of the art leads to opportunities and challenges. Deep learning now allows the detection, classification and prediction of spatiotemporal patterns in continuous and discrete data. This allows for a functionality that is now called Cognitive Computing, aimed at uncovering meaningful knowledge from raw data. However, there are also several challenges. First, it should be noted that current neural-networking methods are implemented on Turing/Von Neuman machines with a very high-energy demand, both for computing and communication functions. At the same time, the human brain, with 9x1010 neurons (1.5x1014 connections), uses less energy than a light bulb. Current GPUs for neural-network training may be 1000 Watts each, and their information-processing capacity is still limited in comparison to the human brain. Notably, a snapshot of electrical brain activity will reveal that most neurons are silent. The neuron can be considered a spike oscillator (point-process generator), firing only when necessary.
To discover and exploit new materials with nonlinearity and adaptive connectivity, it is necessary to determine the fundamental classes of computing that are suitable candidates for neuromorphic computing, using both mathematical theory and simulation. Finally, despite the success of deep learning, there are theoretical and practical challenges: How do we prevent and detect inappropriate instances of learned models? How do we learn from raw data with a minimum of training examples? In close cooperation with the materials scientists, we will develop models and methods facilitating the search for materials that are suitable for neural computing due to their electrical nonlinearity and ability for trace formation. In this way, we can cover the full range from low-level modelling to cognitive principles. Our strong international connections with researchers at the CWI (Centrum voor Wiskunde en Informatica) in Amsterdam (Sander Bohte) and at the University of Waterloo (Chris Eliasmith) are highly beneficial in this endeavour.
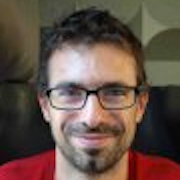
Prof. dr. Davide Grossi
Cognitive Multiagent Systems
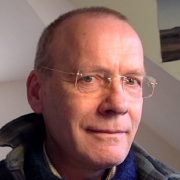
Prof. dr. Herbert Jaeger
Computation in Cognitive Materials
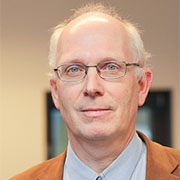
Prof. dr. Lambert Schomaker
Artificial Intelligence
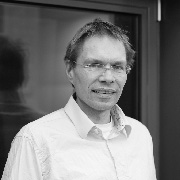
Prof. dr. Niels Taatgen
Artificial Intelligence
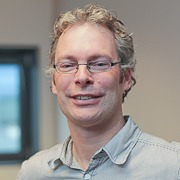
Prof. dr. Bart Verheij
Computational argumentation
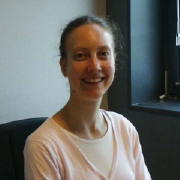
Marieke van Vugt
Cognitive Modeling
Last modified: | 02 February 2024 1.50 p.m. |