Artificial Intelligence makes decisions in uncertain situations
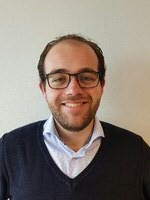
Policymakers often have to base their decisions on very limited information. Some of these decisions may have a serious impact on society in the future. The world of small business is also prone to uncertainty when making decisions, despite potentially huge consequences for both business owners and customers. As a postdoc researcher in the Operations Department of the Faculty of Economics and Business (FEB), Albert Schrotenboer* conducted research in the field of Operations Research (OR). He used mathematical decision models and artificial intelligence (AI) to optimize decisions in which uncertainty plays a large role.
Text: Bart Talens/Industry Relations UG
‘OR is about optimizing decisions. That sounds very general, and it is. You can imagine that it is difficult to make good decisions about isolated or abstract objects, or about things that are already running, that cost money, or that could be made more efficient. In OR, we try to optimize this process,’ explains Schrotenboer. The main goal is, in principle, to make the optimum decision. ‘AI is a hot topic at the moment but we’ve been using similar methods in OR for years. They just haven’t hit the headlines, I suppose.’
Identifying links
In his research, Schrotenboer uses mathematical models, simulations and reinforcement learning to develop methods for making the best possible decisions despite uncertainty. ‘In cases like this, you tend not to go for the optimum decision but instead, you try to find the best possible decision. This is where AI comes in. It allows you to work with huge datasets and to identify links that would be impossible to find without AI or optimization,’ Schrotenboer explains.
Uncertainty regarding parcels
Schrotenboer himself is involved in research into Dropper, a bicycle courier service that aims to deliver parcels from A to B in the city of Groningen within a day. ‘This is a good example of a very uncertain situation in the business world. You never know when you will be presented with parcels or which routes will be used. In addition, you have to take external factors such as the weather and traffic into account when planning your deliveries.’ He has now built a model that simulates Dropper’s business processes. The model uses decision-making rules, which state where and when the bike couriers can be deployed, to evaluate the efficiency of Dropper’s business processes.
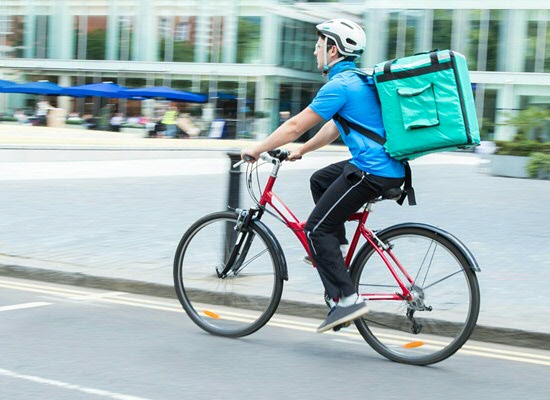
Streamlining courier routes
Based on these simulations, Dropper can make decisions about when to send a courier out on a delivery. ‘If a parcel is registered in Beijum, for example, it might be better to wait before sending a courier out. Half-an-hour later, you might have another parcel for delivery on Korreweg, which is on the same route, or there could be another courier out on a delivery, whose route is more convenient for that particular parcel.’ Reinforcement learning, the process of learning the results of decision-making rules in the simulations, is helping Dropper to streamline its deployment of couriers.
Common sense
Schrotenboer says that when using methods like this, it is important to get to know the company involved. It is a big step for some companies to entrust their working methods to a mathematical model. Furthermore, a simulation can never account for underlying human factors in the workplace. So, it is a process whereby a computed model must go hand-in-hand with ‘old-fashioned common sense’, as Schrotenboer puts it. ‘In theory, you could do this work remotely if you had a run-down of ongoing problems and access to the right data. But certain factors, although unrelated to the essence of a problem, may still have a huge influence on a process in practical terms.’ Relationships between people in the workplace, for example, are a prime example.
Setting up a hydrogen chain
Schrotenboer is now also involved in HEAVENN, the Hydrogen Valley that is set to make the Northern Netherlands a testing ground for setting up a fully functioning green hydrogen chain. ‘Uncertainty obviously plays a role at innumerable levels in a project like this. Political, technological and economic factors can be important.’ Schrotenboer cites the modifications to the current electricity infrastructure and the real-time matching of energy consumption in the region as examples of factors in which OR can play a large role. ‘Unlike the Dropper project, you can’t get as close to the practical situation in these projects. Analysing the available data and devising the model are a much larger part of the process.’ OR involves two separate steps. First, the methodology, developing the models and then applying the models in practice.
So, within OR, AI is used to formulate solid solutions to decision-making problems in which uncertainty plays a large role. AI does not make the final decision but serves as a tool that helps people to make that final decision.
* Since January 2021, Albert Schrotenboer has been working as an Assistant Professor of Transportation and Logistics in the School of Engineering at Eindhoven University of Technology.
Last modified: | 22 January 2021 09.09 a.m. |
More news
-
25 April 2024
Lineke Sneller appointed as Professor of Practice of Digitalization & AI in Accounting and Auditing
The Faculty of Economics and Business (FEB) is pleased to announce that as of 1 May, professor Lineke Sneller will be appointed as Professor Practice of Digitalization & AI in Accounting and Auditing. The chair is situated within the department of...
-
25 April 2024
Jenny van Doorn and co-authors receive 2024 Weitz-Winer-O’Dell Award
Jenny van Doorn and co-authors Martin Mende, Maura L. Scott (both Florida State University), Dhruv Grewal (Babson College) and Ilana Shanks (Stony Brook University) have won the 2024 Weitz-Winer-O’Dell Award. They received the award for their paper...
-
19 April 2024
New thesis prize for master's students of Economics and Business
How can we encourage economics and business students to deal with important societal challenges in their master's thesis? The 14 Dutch faculties of economics and business, united in the Council of Deans in Economics and Business (DEB), have set up...