Which economic forecasts do we trust?
Date: | 26 November 2021 |
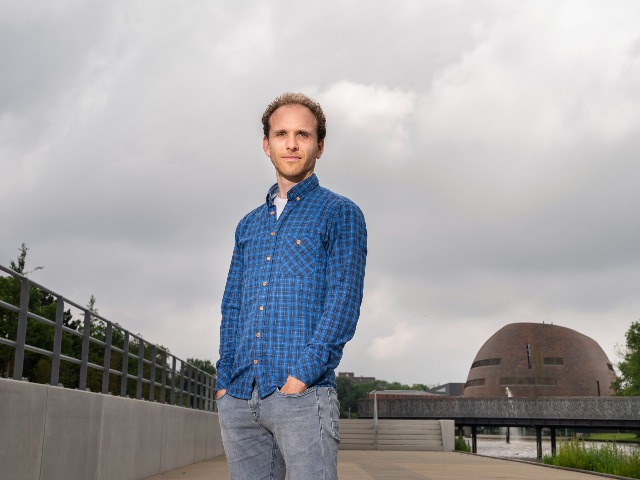
At the end of last year, dr Tom Boot, dr Christiaan van der Kwaak and dr Björn Mitzinneck received a Veni grant from the Dutch Research Council ( NWO ) worth up to €250,000. The grants provide the laureates with the opportunity to further develop their own research projects during a period of three years. The VENI grants are aimed at excellent researchers who have recently obtained their doctorate.
"I first came to the Zernike campus in 2004. Back then, instead of taking a left towards the Duisenberg building, I took a right to the pinnacle of Groningen architecture, the physics building. Architecture-wise, the only way up from there was to move to Rotterdam, where I completed a PhD in Econometrics in 2016. I was very happy to find a position back in Groningen, where I am now associate professor."
My Veni research
"In the past year, we have seen many, often contradicting forecasts on the economic consequences of the Covid pandemic. Even professional forecasters tend to disagree quite strongly. As an example, Figure 1 shows the forecasts provided in the ECB Survey of Professional Forecasters (SPF) in the survey round of 2020Q2 for year-on-year real GDP percentage growth in the euro area for 2020Q4. The large spread in Figure 1 raises the question: who do we trust here?
One way to decide who we trust is to measure which institutions or experts on average have provided accurate forecasts in the past. However, given the unique nature of the Covid crisis, what’s the value of such average past performance? Shouldn’t we adapt our forecast selection to today’s circumstances? In 2008 we might want to listen to experts on the financial system, while in 2020 we rather focus on forecasters with expert knowledge on health economics.
Adapting forecast selection to current conditions is challengingly interesting, both empirically and theoretically. Empirically, in order to decide who’s an expert given today’s conditions, we need to measure forecast performance in time periods `similar’ to today. How do we measure such similarity? Macroeconomic databases contain thousands of economic indicators that can be used to find similar time periods. Using all of them most likely leaves us with an empty set: there was no past time period exactly like the one in March 2020. On the other hand, subjectively selecting a few indicators makes it easy to miss important factors that are not on our radar.
My research will use techniques that some refer to as machine learning algorithms, to automatically find that part of the available data set that is most relevant to assess how well a forecaster is going to perform in today’s circumstances. A key element of the research is to understand how these algorithms are able to find the relevant data. One concrete component is to further uncover a deep connection with traditional factor models -- which suppose that a small number of latent variables drives most variation in the observed data -- for which we developed some initial ideas in [2].
Being a theoretical econometrician, I would also like to study the properties of the forecast that we select based on our conditional evaluation. This is not just for academic purposes. If we want to display forecast uncertainty, we need to know the forecast error distribution. For simple time series models, it is known that the distribution of forecast errors changes when we condition on the current observation. This effect is particularly strong when the current observation is more extreme (again, enter the Covid crisis). The second part of my research will focus on extending these ideas to the modern empirical setting where current conditions are not accurately described by a single variable, but rather by a large set of potentially relevant variables that we find in modern databases."
Societal relevance
"In the past year, short-term forecasts on medical numbers such as ICU occupation, as well as economic indicators such as unemployment, have been an important factor in government decision making. The CPB, responsible for making the economic forecasts, has recently started to work with machine learning methods to improve their procedures. Given the importance of the decisions that are based on these forecasts, we need to know when (and why) these methods will be accurate and when they will not. I recently presented some of my research at a CPB data science symposium that helps to understand why the models they use in fact `work’ in macroeconomic forecasting. I’m looking forward to three years of opening these black boxes so that they become more attractive and reliable for practical use.”
Key publications
Boot, T. and Pick, A. (2020). Does modeling a structural break improve forecast accuracy? Journal of Econometrics, 215(1):35-59
Boot, T. and Nibbering, D. (2019). Forecasting using random subspace methods, Journal of Econometrics, 209(2):391-406
Boot, T. and Pick, A. (2018). Optimal forecasts from Markov switching models, Journal of Business & Economic Statistics, 36(4):628-642.