Predicting Vocal Aging with Machine Learning: A Voice for the Future
Date: | 14 May 2025 |
Author: | Hiva Naazeri |
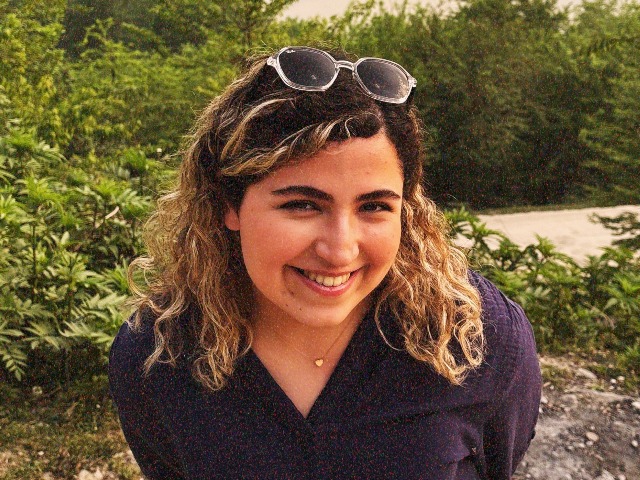
Imagine being able to tell someone’s age just by listening to their voice. Our voices naturally change as we grow older, with changes in how strong or clear it sounds. These changes are more than just natural effects of aging on the voice— they hold valuable information that can be used in healthcare, forensic investigations, and human-computer interaction. My research explores how machine learning can analyze these vocal shifts to predict age and, potentially, detect age related speech disorders earlier.
Why Study Voice Aging?
As we age, our vocal cords and respiratory system experience transformations that affect how we sound. You might notice that older adults often have slightly weaker, breathier, or deeper. This isn’t just perception—it’s based on measurable shifts in key acoustic features like:
▪ Fundamental frequency (F0): The pitch of the voice tends to lower with age.
▪ Jitter and shimmer: These indicate voice stability, which often decreases over time.
▪ Formant frequencies: Changes in resonance due to vocal tract adjustments.
▪ Spectral tilt: A measure of how energy is distributed across different frequencies.
By training machine learning models to recognize these patterns, we can develop tools to assess vocal aging with greater precision.

How Does Machine Learning Help?
Traditional studies relied on manual analysis of voice recordings, which is time-consuming and inconsistent. Machine learning automates and improves this process by identifying subtle acoustic patterns across large datasets. My research focuses on training models with a minimal yet effective feature set—this means finding the perfect balance of parameters to achieve high accuracy without unnecessary complexity.
Using datasets from both healthy and disordered speakers, I’m testing different algorithms to predict speaker age with at least 85% accuracy. The goal is not only to enhance scientific understanding but also to create practical applications, such as:
▪ Early detection of voice disorders: Conditions like Presbyphonia (age-related voice weakness) could be identified sooner, allowing for timely intervention.
▪ Forensic applications: Age estimation can aid criminal investigations and identity verification.
▪ Improved voice-assisted technologies: Speech recognition systems could adapt better to elderly users, making digital assistants more accessible.
Bridging Science and Real-World Impact
One major challenge in this research is ensuring that models work across different languages and voice types. Voice aging varies between individuals based on factors like gender, health, and linguistic background. My study addresses this by selecting diverse datasets and applying feature selection techniques to optimize accuracy.
The next step? Refining the model to improve efficiency and testing its performance on new voice samples. If successful, this research could lead to advancements in healthcare diagnostics, assistive technology, and even the way we interact with voice-based AI.
Science isn’t just about discovery—it’s about making life better. By unlocking the secrets of vocal aging, we can give people a clearer voice in their later years, on both a practical and symbolic level.
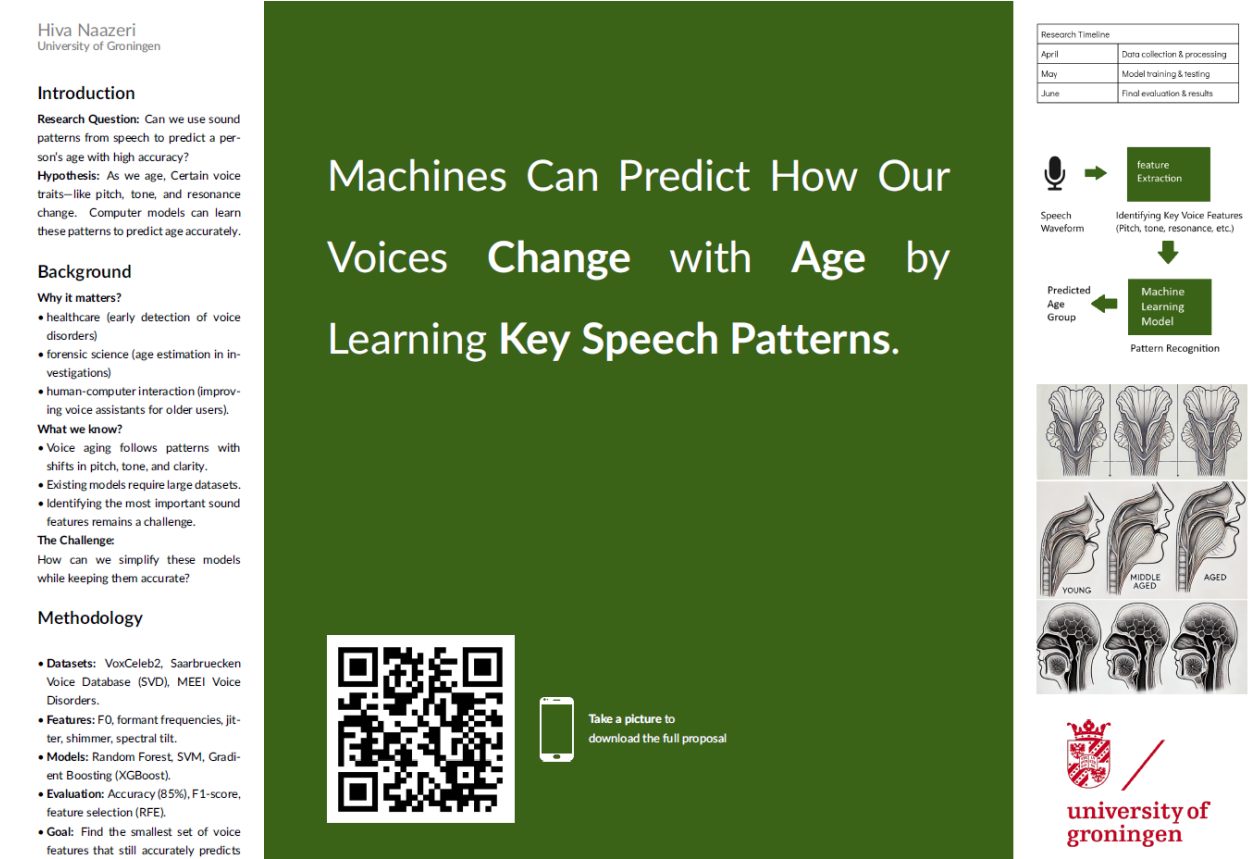
Join the programme and be next in line for an inspiring opportunity!
Visit our MSc Speech Technology webpage and sign up for our monthly newsletter. See you there!
About the author
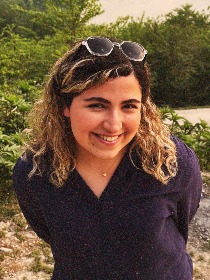
Hiva Naazeri is a student of the MSc in Voice Technology (now Speech technology) at Campus Fryslân, University of Groningen.