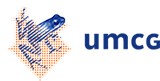
Gerton Lunter
Gerton Lunter is professor of Medical Statistics at the University Medical Center Groningen (UMCG). He is head of the unit 'Medical Statistics and Decision Making' at the Department of Epidemiology. His expertise includes Bayesian statistics, statistical genetics, bioinformatics and applications of machine learning.
After obtaining his PhD in mathematics (Groningen, 2000) Gerton briefly worked at Philips Research (Eindhoven) before moving to Oxford (UK) to focus on bioinformatics and evolutionary genomics. He contributed to the 1000 Genomics Project, and developed various software tools for DNA sequence analysis. In 2014 he co-founded Genomics PLC, and in the same year was appointed Associate Professor and Group Leader at the Wellcome Centre for Human Genetics (Oxford). In 2019 he joined the Weatherall Institute of Molecular Medicine (Oxford) as Group Leader in Computational Biology and Artificial Intelligence, where he is currently appointed as Visiting Professor.
His research interest is in designing statistical algorithms to extract knowledge from large datasets. His current focus is on developing statistical models for large and high-dimensional longitudinal cohorts, such as Lifelines. Ongoing research interests are using deep learning to predict genomic phenotypes from sequence, including splicing, chromatin accessibility, and gene expression. Such models help us to better understand genome biology, improve our interpretation of genetic association studies, help clinicians to make diagnoses involving rare genetic mutations, and ultimately improve health outcomes.
Gerton has (co-)supervised 10 PhD projects, published 77 papers (Scopus H index, 42) and is named on 9 patents. He organized two international meetings, and is regularly invited as program committee member of international conferences.
Key publications
D P Cooke, D C Wedge, G Lunter (2021). A unified haplotype-based method for accurate andcomprehensive variant calling. Nat Biotech https://doi.org/ 10.1038/s41587-021-00861-3
R Schwessinger, M Gosden,D Downes, R Brown, AM Oudelaar, J Telenius, YW Teh, G Lunter, JR Hughes (2020). DeepC: predicting 3D genome folding using megabase-scale transfer learning. Nat Methods 17, 1118–24.
R Brown, G Lunter (2018). An Equivariant Bayesian Convolutional Network predicts recombination hotspots and accurately resolves binding motifs. (2018) Bioinformatics bty964
WGS500 Project Consortium (2015) Factors influencing success of clinical genome sequencing across a broad spectrum of disorders. Nature Genetics 47(7) 717-26.
A Rimmer A, H Phan, I Mathieson, Z Iqbal, SR Twigg; WGS500 Consortium, AO Wilkie, G McVean, G Lunter (2014) Integrating mapping-, assembly- and haplotype-based approaches for calling variants in clinical sequencing applications. Nat Genet. 46(8), pp. 912-8
Last modified: | 12 August 2024 12.35 p.m. |