How can universities support open science?
Date: | 17 October 2018 |
Author: | Giulia Trentacosti |
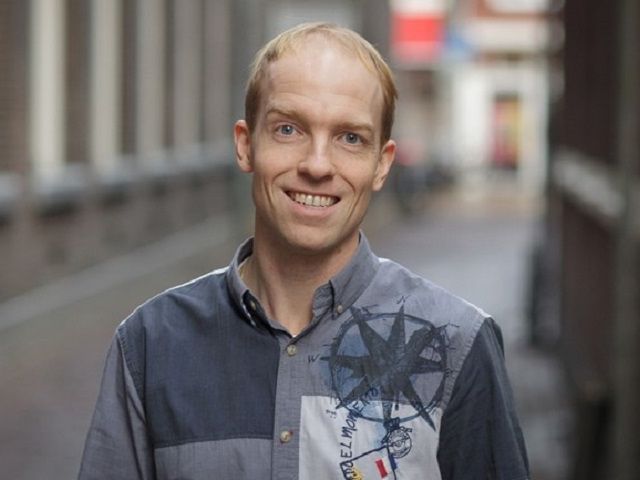
How can universities support open science?
An interview with Martijn Wieling
The Open Science movement advocates a cultural shift towards openness and transparency in all stages of the research process. It does so by promoting open access to scientific publications, by encouraging data and source sharing, by calling for the implementation of improved criteria in research evaluation, and by placing a strong emphasis on citizen science and public engagement.
In this interview, Associate Professor of Computational Linguistics and Vice-chairman of the Dutch Young Academy, Martijn Wieling, discusses his take on open science, the role of higher education institutions, and why he thinks open access, open data and open source are the way forward in academic research.
'To really make the transition to open science, I think it is essential that the universities invest in making this possible. Concretely, that would mean reducing the teaching or administrative workload in order to allow researchers more time to share research data and code adequately.'
What is the level of uptake of open access in computational linguistics?
Open access is the dominant way of publishing in our field. We publish in conference proceedings through the Association for Computational Linguistics (ACL). In short, you pay the conference fee to attend ACL conferences and then the proceedings are published open access for free. In addition, the prominent journals, Computational Linguisticsand Transactions of the ACL, are also open access.
What about open data? Would you say it is common practice to share data in your field?
In computational linguistics most of the data is openly accessible – I would say probably 80%. The reason for this is that we often use standard data sets to carry out our research and usually these data sets are freely available (although sometimes you have to pay a fee to access them). However, in my field, we often use custom-made software to analyse the data. Consequently, sharing the underlying code is even more important. Publications in computational linguistics papers are usually 8 pages long – that is the maximum length for publications in the conference proceedings – and that is generally insufficient to discuss all the details. If the code is shared, others can look into the details and reproduce the study, which I think is essential.
Source code for these programs is increasingly shared. While I have the feeling that this is improving, we are not there yet. A while ago, two colleagues and I did a study, which was recently published in Computational Linguistics. In this study, we looked at all the papers presented at the 2011 and 2016 annual ACL conferences to establish whether the data and the source code were publicly available. First, we read through the papers, checked whether there were links to the data and, if so, whether these links were still working. If the data or source code was not accessible, we contacted the authors asking them to provide us with the necessary data and source code to reproduce the results of the study. For 2016, we obtained the underlying source code in about 60% of the cases, while for 2011 we could only do so for 33%. Of course, 2011 is a longer time ago – in the meantime, people may have moved universities, hard drives may have crashed, and so on. While I think these results are not too bad compared to other fields, I think we should do better, since it is fairly easy to share data and source code in our discipline.
I also want to note that data sharing is not feasible for all fields. In the humanities, it is sometimes very difficult to define what data is. For example, if you write about literature, are your notes the data which you should share? Alternatively, in some fields in the natural sciences, experiments may generate petabytes of data. In these cases, you could make the data open, but nobody would be able to download it, which means that ultimately the data is still not accessible. Another reason why researchers might be prevented from sharing data is that sometimes studies are conducted in collaboration with companies, which often do not agree to share the data or the code. Then, of course, there are cases of studies where you have to consider very carefully whether sharing data is desirable, particularly when there are patients involved. Even if you anonymize the data, you might still be able to identify the participants. While I am a great proponent of open data, I do not think that data should be open at all costs.
What do you think are the main obstacles to open science and what do you think institutions can do to overcome these obstacles?
I think there are quite a few obstacles. The main thing that needs to change is people’s mind set. Researchers need to become more aware of the need to share their data, and they should start to integrate this practice in their workflow. Having to make your data open after you have already published a paper usually takes more effort. If you integrate this into your research workflow, and do it at an earlier stage, you can usually save a great deal of time, which, in turn, might increase the uptake of such an approach.
For example, I was once asked for some software that I wrote and used in a study a few years ago, but had not made publicly available. Subsequently, it took me quite some time to find it and figure out how it worked. I think the goal of open science should be to set up the research project in such a way that if you look at your data in 5 years’ time , you are still able to understand your steps. Of course, you should also make sure that others are able to reproduce your analysis by using the same procedure.
Concerning the role of institutions in facilitating open science, I think that sharing data should be made as easy and straightforward as possible for researchers. If it requires effort, researchers are less likely to do it, since they are already more than busy enough. I think it would be best if we could share our data directly via an interface in Pure, without having to also login at another repository (e.g. DataverseNL). When I upload my publication to Pure, I would also like to be able to upload the associated research data, including a description of the data and the procedures used to analyse it. The publication and the data are intimately linked, and I think ideally one should be able to publish them simultaneously using one platform.
While young researchers are now often trained in these topics from the start of their career, more senior researchers are not always accustomed to the practice of open science. I think that institutions should put more effort into incentivizing and rewarding good practices of open science. Academics are very busy and sharing research data is an additional task. Of course, we all know that making your research data open is a good thing, but we also know that often there are not many people who will download the data associated with a single paper. While there are some prizes that reward attempts to make data open, I do not think that this will stimulate open science enough. Specifically, since making your data open does not really help in grant applications, but does require time (which you cannot spend on writing another publication), if open science became a more important criterion when evaluating research proposals, then people would probably share their data more often. Then again, this would add yet another box for researchers to tick. To really make the transition to open science, I think it is essential that the universities invest in making this possible. Concretely, that would mean reducing the teaching or administrative workload in order to allow researchers more time to share research data and code adequately.
Finally, I think institutions should not be overly strict about what format should be used when uploading data into data repositories. For me open data and open code means that you have to provide the data and instructions that allow someone else to re-use it. If sharing data is too complicated and it takes too much time to adhere to certain standards, researchers will simply not do it.
What do you do to educate PhD and postdocs about these issues?
When I teach courses in statistics, I always ask students to conduct a reproducible analysis. We generally use the open source statistics program R for this, through which it is possible to generate a research report containing both the code and the results. This means that after running the analysis you can share the report generated, and everybody can run the same analysis if you provide them with the underlying data. I think it is important that students learn this as soon as possible, and therefore I teach students from the first year of their studies onwards to use this approach.
You are the Vice-chairman of the Dutch Young Academy. Can you tell us a bit more about the YA’s position with regard to open science?
The Dutch Young Academy wholeheartedly supports open access and open science because we think that research which is funded with public money should be publicly available. Nevertheless, the Dutch Young Academy also acknowledges differences across disciplines and therefore we believe that whether data should be shared depends on the specific field. While the results of scientific research should ideally be available through open access, some disciplines (especially in the humanities) publish in books which lend themselves less well to open access publishing. So, open science where possible, but other solutions where necessary.