New in Groningen: Weining Wang
Date: | 01 February 2024 |
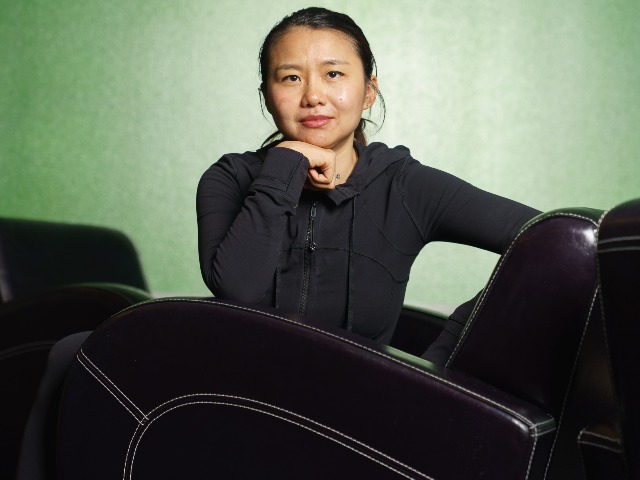
Weining Wang recently joined the Faculty of Economics and Business as Professor of Econometrics. Before coming to Groningen, she worked at various universities in Europe: in Germany and the United Kingdom. Her research focuses broadly on financial and theoretical econometrics. More specifically, Wang works with modern machine learning methods and their applications in finance. In her new role as professor at FEB, she strives to enhance and further integrate data science research.
Why did you choose Groningen?
“I chose to come to Groningen because the University of Groningen is a prestigious university; it is the second oldest university in the Netherlands and has a high reputation within Europe. When it comes to my field of expertise, economics and econometrics, the Faculty of Economics and Business in Groningen is a renowned institute with world-class research.”
Could you tell us about your career so far?
“I obtained a PhD at the Humboldt University of Berlin in 2012 and continued there as a junior professor. Subsequently, I moved to teach at City University of London as a tenured Associate Professor. In 2019, I became a Chair Professor in Financial Econometrics at the University of York. In September of 2023, I moved to the University of Groningen. Over all these years, my research had broadly been focused on financial and theoretical econometrics. Apart from carrying out research in these fields, I have also gained wide experience in teaching econometrics, statistics, micro-econometrics, finance and programming”
Your chair is in Econometrics. What kind of issues are dealt with in your research?
“My research concerns developing sophisticated econometric methodologies to improve decisionmaking in the field of finance and other related fields. In the years to come, I would like to build econometrics and machine learning tools for understanding the economic and social value of cryptocurrencies within the financial market.”
What is the societal relevance of your research?
“I work with modern machine learning methods and their applications in finance. There is a strong demand for developing more data-driven techniques that allow market participants to interrogate their sources in an informative, accurate, and flexible manner. The massive sample size, high dimensionality, and complex dependence in the financial system, create computational and statistical challenges in the network data that cannot be handled by conventional decision analytical methods.
In the scientific investigation of individual behavior and market reactions, econometric network analysis plays an increasingly important role. On the other hand, in these typically network-organized data, the amount of diversion and diffusion inherently creates risk structures that require additional scientific insight to be practically relevant in decision analytics. Connectedness of networks, for example, is central to modern risk measurement since it proxies market risk, credit risk, and systemic risk. Not surprisingly, such network risk has been based on correlationbased measures, hence closely connected to linear Gaussian thinking, making strict inferences of limited value in many applications.
My work also studies the estimation and inference of network connectedness for high-dimensional time series. However, this does not concern uncovering latent or mis-specified linkages. With colleagues, I look at tail event networks (TEN) that reflect the dependencies of vertices (players, nodes, institutions, cells) in stress-dominated situations. In previous research, we assessed the tail interconnectedness of a single financial institution with all other financial companies simultaneously. The primary challenge was selecting the set of relevant risk drivers for each financial institution. Statistically we addressed this issue by employing the a variable selection method in the context of a single-index model for generalized quantile regressions. We further extended it to a time series variable selection context in ultra-high dimensions. Further, we proposed two indices, namely, systemic contribution index and systemic exposure index where we ranked all the companies based on their degree of their contribution (or exposure) to systemic risk.
The model was evaluated based on daily return data on 200 publicly traded US financial institutions from January 1, 2006 till September 1, 2012 This provides useful rankings of systemic exposure and systemic contribution at various stages of financial crises. Network analysis, its behaviour and dynamics, allows us to characterize a role of each sector in the financial crisis and yields a new perspective of the US financial market 2007 - 2012”
What can we expect from you in the future?
“I will continue to contribute to the research excellence of FEB, primarily in three aspects: First, I will continue to generate world-class research output and collaborate more with junior faculties and students at FEB. Second, I will help enhance the research environment by organizing seminars, webinars, and research conferences. I will strive to integrate into the Data Science Research Centre and improve the academic standing of Groningen in data science research. Third, I will try to collaborate with researchers across fields to generate more research income and research impact.”
For more information: