Big data verkennen in de astronomie: een interview met Gijs Verdoes Kleijn en Rees Williams
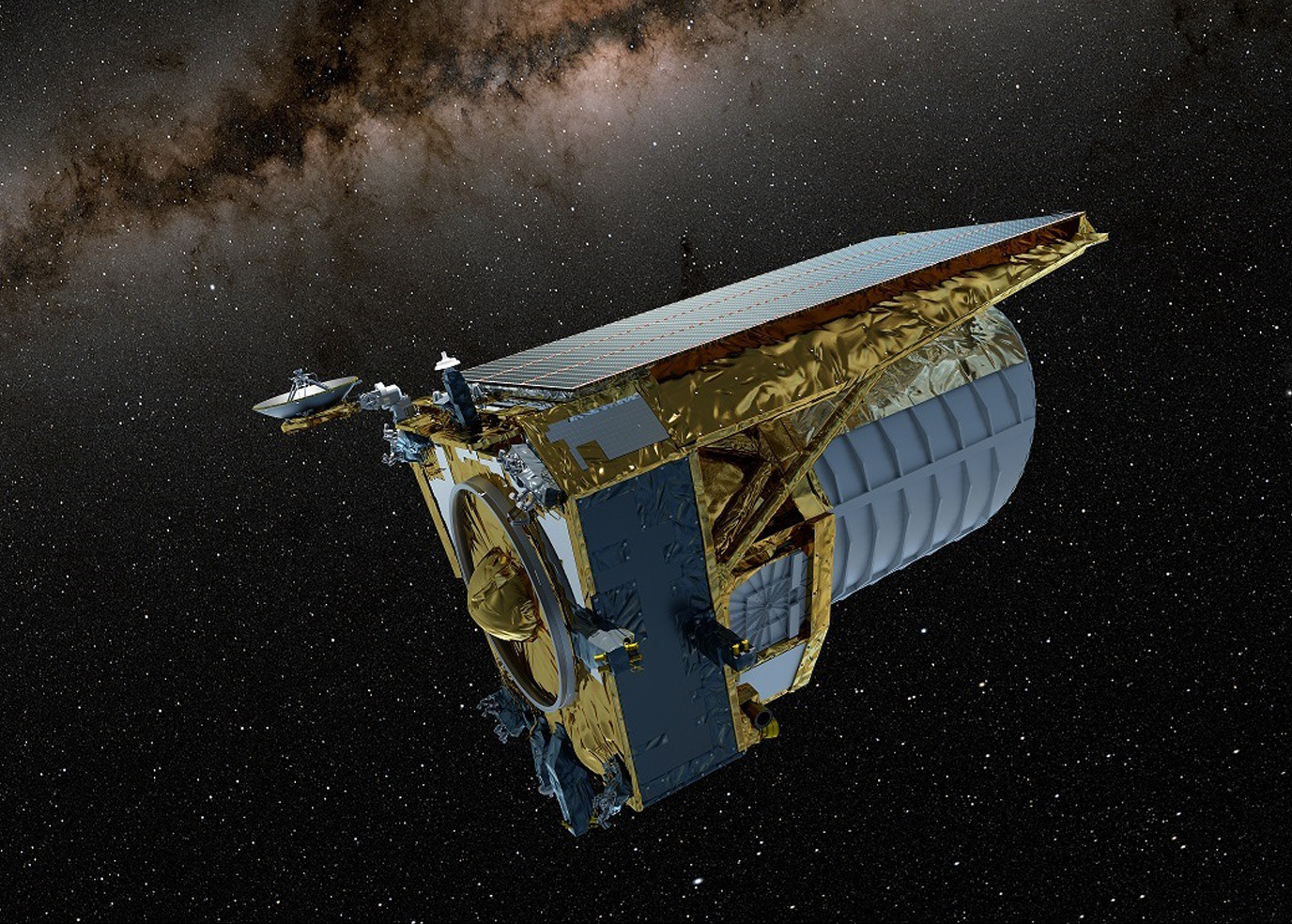
In het vakgebied van de astronomie is het verzamelen en analyseren van enorme datasets van doorslaggevend belang geworden voor het ontdekken van de geheimen van het heelal. Gijs Verdoes Kleijn en Rees Williams houden zich bezig met data-intensieve astronomie en brengen elk een schat aan ervaring en expertise mee. Dit interview laat zien dat hun gecombineerde kennis leidt tot inzichten van onschatbare waarde in de uitdagingen en innovaties op het gebied van het beheren van astronomische gegevens.
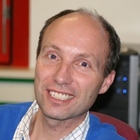
Gijs Verdoes Kleijn is een astronoom die zich bezighoudt met big data bij het Kapteyn Instituut, waar het OmegaCEN Astronomical Science Data Centre, is gevestigd, en hij staat bekend om zijn werk op het gebied van de astronomische datawetenschap. Zijn onderzoek richt zich op het zoeken naar exotische objecten in onmetelijke oceanen van astronomische gegevens.
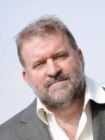
Rees Williams, projectmanager bij het Centrum voor Informatie Technologie (CIT) van de RUG en OmegaCEN, heeft meer dan 35 jaar ervaring in het verwerken en archiveren van gegevens afkomstig van op satellieten gemonteerde astronomische instrumenten. Zijn expertise omvat röntgen-, gammastralings- en optische instrumenten, en zijn meest recente project betreft de Euclid-satelliet.
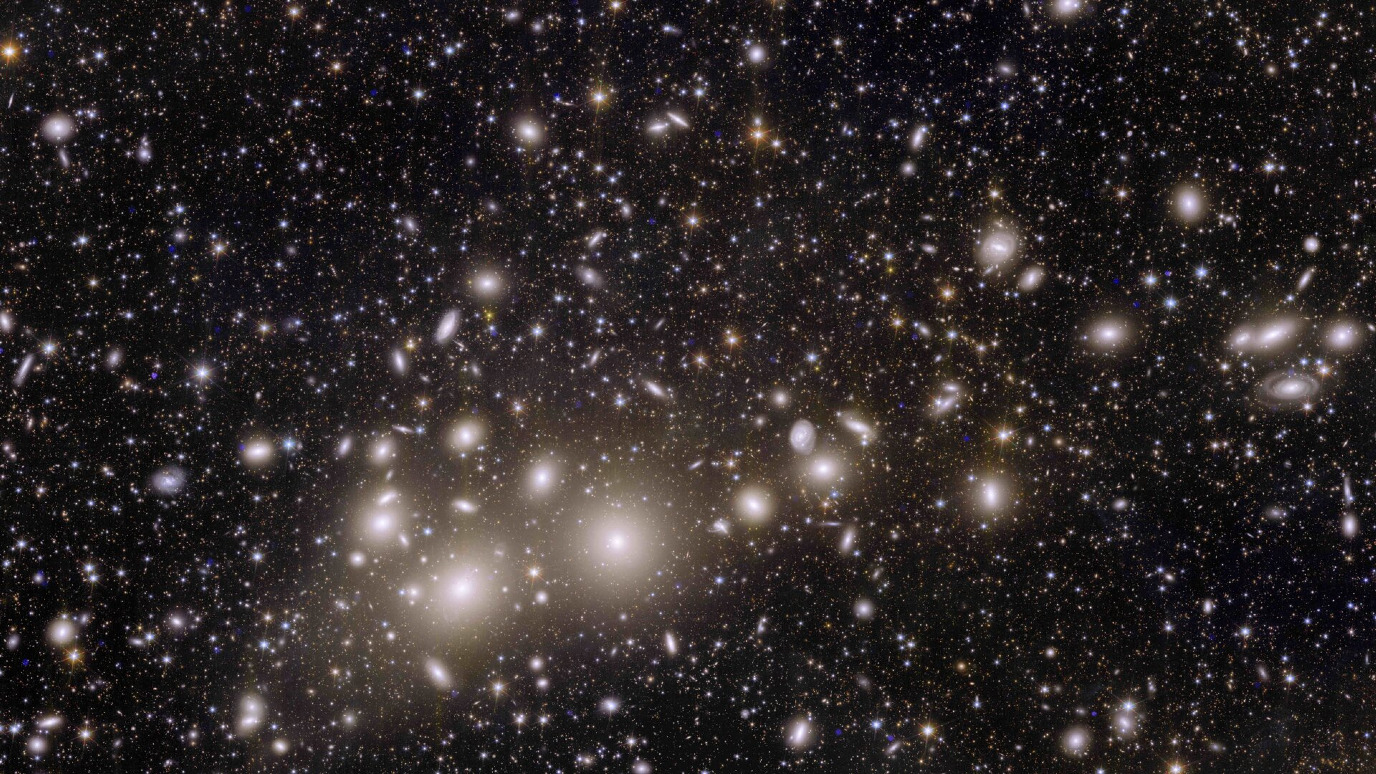
Het is niet alleen de hoeveelheid die het tot big data maakt; het is ook de complexiteit.
Met welke soorten big data werk je meestal in je onderzoek?
Gijs: Ik werk meestal met beeldgegevens. Hierbij gaat het om het verwerken van ruwe datavolumes variërend van een paar honderd terabytes tot petabytes. Het is niet alleen de hoeveelheid die het tot big data maakt; het is ook de complexiteit. De beelden moeten uitvoerig worden geanalyseerd en verwerkt om ze zo te kalibreren dat ze geschikt zijn voor wetenschappelijk onderzoek. Daarna wordt de informatie over de wetenschappelijke analyse opgeslagen in een database, waardoor een rijke bron aan astronomische informatie ontstaat.
Rees: Het gaat niet alleen om de hoeveelheid, maar ook om de complexiteit en de veranderlijkheid van de gegevens. Bij projecten zoals Euclid zijn de datasets bijvoorbeeld zeer complex en moeten ze vaak worden bijgewerkt en opnieuw worden gekalibreerd.
Gijs: Wat Rees zegt, klopt. Bij sommige projecten kun je gemakkelijk opnieuw een database maken als je een betere manier hebt gevonden. Maar als je werkt met terabytes aan metadata die in de loop der jaren zijn verzameld, kun je die niet zomaar weggooien. Je moet in dat geval gegevens uit het verleden zorgvuldig bewaren terwijl je nieuwe inzichten toevoegt, en dat vraagt om een hoge mate van wendbaarheid en flexibiliteit van je database om de ontwikkelingen te kunnen volgen.
Over wat voor soort databases hebben we het?
Gijs: Wij gebruiken relationele databasemanagementsystemen.
Rees: Inderdaad. Onze database is opgebouwd vanuit een klassieke relationele structuur, maar kan zich ook gedragen als een objectgeoriënteerde database, afhankelijk van wat we nodig hebben. Deze dubbele functionaliteit stelt ons in staat om op een meer natuurlijke manier met gegevens om te gaan en ze te bevragen, omdat astronomen vaak interactie nodig hebben met gegevensobjecten zoals sterren of sterrenstelsels.
Voor het onderhouden van een database die wereldwijd voor iedereen toegankelijk is, zijn aanzienlijke financiële middelen nodig.
Zijn deze databases open toegankelijk?
Rees: De eindresultaten zijn openbaar, maar het hele databasesysteem is meestal niet volledig publiek beschikbaar vanwege financieringsbeperkingen.
Gijs: Ik ben betrokken geweest bij projecten waarbij de eindresultaten werden geëxporteerd om volledig publiek beschikbaar te zijn, zoals bij de Europese Zuidelijke Sterrenwacht. Volledige publieke toegang tot de database is echter alleen mogelijk met voldoende financiering die een dergelijk open systeem mogelijk maakt. Voor het onderhouden van een database die wereldwijd voor iedereen toegankelijk is, zijn aanzienlijke financiële middelen nodig.
Rees: Vanuit mijn achtergrond met satellietdatabases, en dan met name Europese satellieten, zijn het de metadata die worden geselecteerd in de open databases die alle gegevens bevatten. Het archief van het Europees Ruimteagentschap (ESA) telt bijvoorbeeld zo’n 30 tot 40 satellieten. Om deze effectief te beheren, is het hebben van een gestandaardiseerde architectuur van groot belang. Hierdoor wordt het onderhoud eenvoudiger en worden de levensduur en toegankelijkheid van de gegevens gewaarborgd.
Tegen welke uitdagingen lopen jullie aan als het gaat om het beheren, archiveren en openstellen van deze grote datasets?
Rees: De grootste uitdaging is om gegevens zodanig toegankelijk te maken dat mensen ze gemakkelijk kunnen gebruiken. In het geval van Euclid zou het bijvoorbeeld bijna onmogelijk zijn om iedereen toegang te geven tot het verwerkingssysteem. In plaats daarvan bieden we toegang via bekende interfaces zoals virtuele observatoria.
Gijs: Een andere uitdaging is om ervoor te zorgen dat astronomen getraind worden in datawetenschappen. Velen van hen zijn expert in de astrofysica, maar weten lang niet altijd hoe ze om moeten gaan met complexe databases. Deze kloof kan ertoe leiden dat zelfs open data moeilijk op een effectieve manier te gebruiken zijn.
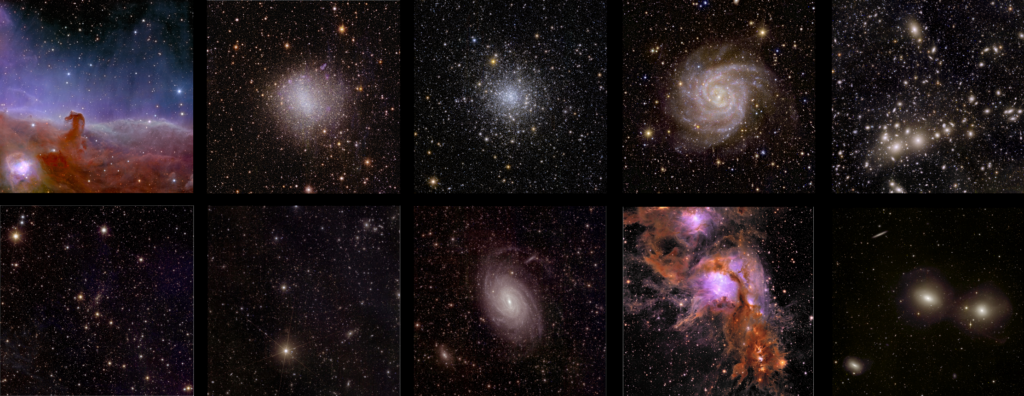
Een ander belangrijk aspect van open data is metadata. Binnen de astronomie zijn metadata gestandaardiseerd. Kun je ons vertellen hoe dit zo’n succes is geworden?
De aard van onze gegevens, die apolitiek zijn en geen privacygevoeligheden hebben, helpt bij de standaardisatie ervan.
Rees: De standaardisatie van databestanden, en in het bijzonder het gebruik van het FITS-formaat, werd wat mij betreft niet echt moedwillig gedaan, maar dit werd uiteindelijk universeel overgenomen. Maar hoewel openbare archieven deze standaarden consequent volgen, doen interne verwerkingssystemen dat vaak niet, waardoor conversie naar het standaardformaat vereist is. Op een vergelijkbare manier zijn metadata gestandaardiseerd volgens de regels van virtuele observatoria, maar ook hier geldt dat dit alleen voor openbare archieven gebeurt en niet voor verwerkingssystemen.
Gijs: Astronomen hebben er goed aan gedaan om standaarden in te stellen, deels omdat onze gemeenschap relatief klein is en ook omdat we op internationaal niveau samenwerken. De aard van onze gegevens, die apolitiek zijn en geen privacygevoeligheden hebben, helpt ook bij deze standaardisatie.
Rees: Ik denk dat het belangrijk is om op te merken dat er slechts een beperkt aantal gangbare gegevenstypen is, waaronder afbeeldingen, spectra, gegevenskubussen en tijdreeksen. Wanneer je echter te maken hebt met ongewone gegevenstypen, zoals gammastralingsexperimenten, worden de gegevens steeds moeilijker om mee te werken. De ruwe gegevens van COMPTEL vormen bijvoorbeeld een vijfdimensionale gegevenskubus: één daarvan is energie, en geen van de overige gegevens biedt de mogelijkheid om een foton te koppelen aan een positie aan de hemel. Dus hoewel de gegevens vindbaar zijn, zijn ze niet langer bruikbaar, en dat is niet in overeenstemming met de FAIR data principes.
Apparatuur is goedkoop, maar mensen duur zijn.
Welke lessen heb je geleerd uit het omgaan met en opslaan van big data?
Gijs: Één belangrijke les is het belang van de afstamming van gegevens, ook wel ‘slakkensporen’ genoemd, die het mogelijk maken om elk stukje informatie terug te traceren tot aan de oorsprong. Als je bijvoorbeeld een resultaat hebt van de morfologie van een melkwegstelsel, moet je weten hoe nauwkeurig dat resultaat is gemeten. Als de foutbalk verdacht is, kun je dagen bezig zijn met het opsporen van de ware oorsprong van de fout. Idealiter zou de database gedetailleerde documentatie moeten verschaffen over hoe elk resultaat tot stand is gekomen, zodat gebruikers elke stap kunnen volgen terug naar de oorspronkelijke gegevens. Het bouwen van zo’n uitgebreide en transparante database is een complexe, uitdagende, maar uiteindelijk dankbare taak die veel tijd en samenwerking vereist.
Rees: Een andere les die ik heb geleerd is dat apparatuur goedkoop is, maar dat mensen duur zijn. Projecten lijden vaak onder pogingen om bestaande, heterogene computertechnologie te gebruiken, wat leidt tot meer complexiteit en hogere kosten. Het is beter om te investeren in uniforme, efficiënte hardware oplossingen, hoewel dit politiek gezien problematisch is.
Hoe waarborg je de kwaliteit en betrouwbaarheid van de gegevens die je verzamelt en analyseert?
Rees: Voor ons huidige project is de grootste uitdaging het waarborgen van de gegevenskwaliteit. Dit vereist aanzienlijke inspanningen en een beoordelingssysteem. Wanneer gegevens worden geproduceerd, moeten ze worden beoordeeld op kwaliteit. Als er problemen worden gevonden, is traceerbaarheid van essentieel belang om de hoofdoorzaak daarvan vast te stellen en aan te pakken. Dit proces wordt steeds herhaald; kwaliteitsmarkeringen alleen zijn niet genoeg.
Gijs: Het waarborgen van datakwaliteit omvat zowel het detecteren als het diagnosticeren van kwaliteitsproblemen. Het detecteren van problemen is vaak eenvoudig, zoals het herkennen wanneer astronomische gegevens niet overeenkomen met verwachte astrofysische patronen. De diagnose van de bron van deze problemen is echter complexer en vereist traceerbaarheid, zoals Rees al aangaf. Zo kunnen kalibratiegegevens bijvoorbeeld worden beïnvloed door niet herkende verschijnselen zoals een zonnestorm, wat tot onjuiste conclusies leidt. Verkeerd geïnterpreteerde signalen kunnen het gevolg zijn van over het hoofd geziene factoren, zoals stof in de Melkweg of fouten in satellietbaancorrecties. Met effectieve traceerbaarheid kunnen deze fouten geïdentificeerd en gecorrigeerd worden om betrouwbare resultaten te garanderen.
Het combineren van grote taalmodellen met onze huidige gegevensinfrastructuur zou een revolutie teweeg kunnen brengen in de manier waarop we sterrenkundig onderzoek uitvoeren.
Welke vooruitgang of ontwikkelingen verwacht je op het gebied van big data management en het delen van gegevens in de sterrenkunde?
Gijs: Ik droom van een toekomst waarin we rechtstreeks kunnen communiceren met gegevensverwerkingssystemen door middel van natuurlijke taal. Stel je voor dat je een programma vraagt om de variabiliteit van sterren in een bepaald gebied te analyseren, dat het programma de taak uitvoert en vervolgens de resultaten interactief bekijkt en verfijnt. Het combineren van grote taalmodellen met onze huidige gegevensinfrastructuur zou een revolutie teweeg kunnen brengen in de manier waarop we sterrenkundig onderzoek uitvoeren.
Rees: Ik zie dat er een behoefte is om gegevens dichtbij het archief te kunnen verwerken zodat ze efficiënter kunnen worden verwerkt. Deze verschuiving vereist veranderingen in financieringsmodellen om internationale samenwerking en het delen van middelen te ondersteunen. Systemen zoals de datalabs van ESA zijn een stap in de goede richting, maar we moeten veel verder gaan. We zouden een model kunnen hanteren dat vergelijkbaar is met supercomputing-centra zoals SURF, waarin onderzoekers bij het gegevensarchief zelf verwerkingstijd aanvragen.
Laatst gewijzigd: | 24 juni 2024 15:49 |
Meer nieuws
-
15 april 2025
Hoe om te gaan met de dataficering van onze maatschappij?
Ons datagebruik is nog nooit zo hoog geweest, maar er ontstaan steeds meer zorgen over onze online aanwezigheid. Wie controleert de online ruimte en hoe wordt dit gedaan? Hoe kunnen overheidsregels helpen om de gevolgen van beslissingen, voor de...
-
05 maart 2025
Vrouwen in de wetenschap
De RUG viert Internationale Vrouwendag met een bijzondere fotoserie: Vrouwen in de wetenschap.
-
07 december 2023
Representing the UG Natural and Engineering Sciences community: an interview with Andrea Capiluppi
Interview with Andrea Capiluppi, associate professor in software engineering at the University of Groningen (UG) who will represent the UG Natural and Engineering Sciences (NES) community within the TDCC-NES governance board for the coming two...